Sydney Business Insights
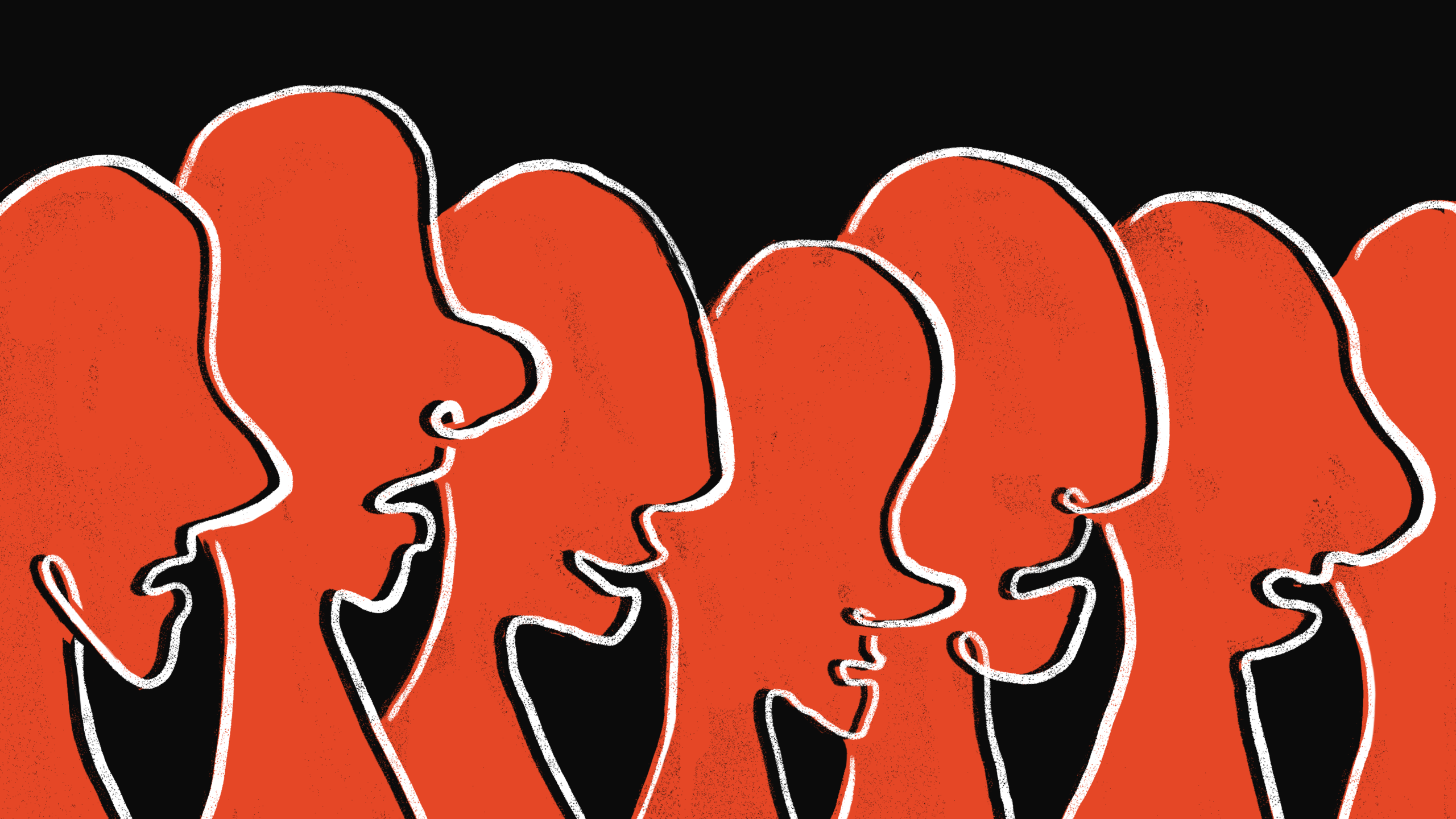
Revisiting Uber, money and monkeys with Keith Chen
As part of our In Conversation series and the University of Sydney’s Business School Global Executive MBA program, we talk to UCLA economist Keith Chen who taught monkeys how to use money in order to better understand how humans make economic decisions.
Show notes and links
University of Sydney’s Business School Global Executive MBA program
Thank you to bbc.co.uk (copyright 2018 BBC) for some of the additional sounds in this podcast.
You can subscribe to this podcast on iTunes, Spotify, Soundcloud, Stitcher, Libsyn, YouTube or wherever you get your podcasts. You can follow us online on Flipboard, Twitter, or on sbi.sydney.edu.au.
Sydney Business Insights is a University of Sydney Business School initiative aiming to provide the business community and public, including our students, alumni and partners with a deeper understanding of major issues and trends around the future of business.
Share
We believe in open and honest access to knowledge. We use a Creative Commons Attribution NoDerivatives licence for our articles and podcasts, so you can republish them for free, online or in print.
Transcript
Sandra: Hi, we're on a break at the moment but here's a conversation we had last year with UCLA's Keith Chen where we discussed behavioural economics, Uber and monkeys.
Intro (audio of Uber drivers, different languages, and monkey noises): Millions of people around the world drive with Uber every single week... I like the freedom about it the flexibility...choose when and where they receive business...a high demand period that Uber calls a surge... if you're not intending on taking rides for a particular period of time... I like driving for Uber... or drive the flat rate and make a lot less money...
Sandra Peter: Keith Chen has studied how monkeys and people including Uber drivers react to financial incentives. He has interrogated grammar rules and savings rates and identified that what language you speak inherently makes you a better saver - or not. If these seem like widely disparate intellectual domains it's because Keith is a behavioural economist which kind of makes him a bit of a David Attenborough of the business world. Keith's work offers insights into: what makes some language groups natural savers; how much money will it take to nudge Uber drivers out to work on a cold dark night and even how far away from our jobs we are prepared to live. He investigates our most base emotions to find out what will humans do for how much money and why sometimes money does not matter at all...
Intro: From the University of Sydney Business School this is Sydney Business Insights. The podcast that explores the future of business.
Sandra: Hello I'm Sandra Peter and in this episode of Sydney Business Insights - monkey money business. As part of the University of Sydney Business School's Global Executive MBA program we met with UCLA economist Keith Chen who taught monkeys how to use money in order to better understand how humans make economic decisions.
Keith Chen: And so actually you know without much of a clue or of a sense of well-founded hopes that this was going to lead to anything. Some colleagues and I began plotting to teach two groups of monkeys how to use money.
Sandra: Not just any sort of monkey - Keith specialises in academic monkeys - the kind whose usual habitat is one of the great US universities. Apparently Yale and Harvard breed different monkey personas.
Keith: One is a small tamarin laboratory at Harvard that has these pair bonding, very social, almost kind of socialist monkeys and the other was these kind of brown tufted capuchins who lived at Yale that were just the opposite of big alpha male society, like very hierarchical, you couldn't ask for two more different groups of monkeys.
Sandra: Behavioural economics is a fairly new branch of the economics tree. Economists like Keith invert traditional economic theory: conventional economics asserts that people make choices based on a rational analysis of what is in their best financial interest. Behavioural economists study people's motivations, be they psychological, cognitive, emotional or cultural, to understand how we make decisions. Moreover they study changes in the man-made environment to see if those adjustments alter our behaviour.
Keith: So one of the things that really jumps out in behavioural economics is that a lot of the most puzzling behaviours we see in people surrounding money and the way that we think about money and the way that we use money is kind of critical metrics and or reference points for a lot of our behaviour.
Sandra: Keith's Ted Talk on how your mother tongue directly influences whether you are a lifelong saver or a spender has been downloaded more than 1.7 million times. As a fluent Chinese and English speaker, Keith understood the two languages treat time - the past, present and future - very differently.
Keith's Ted Talk: This led me, as a behavioural economist, to an intriguing hypothesis. Could how you speak about time, could how your language forces you to think about time, affect your propensity to behave across time? You speak English, a futured language and what that means is that every time you discuss the future, or any kind of a future event, grammatically you're forced to cleave that from the present and treat it as if it's something viscerally different. Now suppose that that visceral difference makes you subtly disassociate the future from the present every time you speak. If that's true and it makes the future feel like something more distant and more different from the present, that's going to make it harder to save. If, on the other hand, you speak of futureless language, the present and the future, you speak about them identically. If that subtly nudges you to feel about them identically, that's going to make it easier to save.
Sandra: Associate Professor Keith Chen is from the nudge school of economics. Nudge proponents advocate the use of incentives to push - or nudge - people into making good decisions. Things like governments automatically enrolling citizens into pension plans, rather than running the voluntary opt-in scheme, have increased the number of people with savings plans. Likewise countries that require their citizens to consciously 'opt-out' of being an organ donor have achieved donor rates over 90 percent. Nations that don't apply this forceful nudge tend to have organ donation rates below 20% of the available population. It's about economically motivating people to do a good thing rather than punishing them for making poor decisions. And companies also use nudge principles to maximise their returns.
Recently Keith has been advising Uber on its pricing policy - helping the ride sharing platform to land on that sweet spot where drivers are making enough money to justify being on the platform ensuring a ready supply, so customers are satisfied with the price and the convenience. But to start with I wanted to know how Keith's monkey studies contributed to his understanding of human behaviour.
Sandra: Keith, you're an economist by training but you are not the traditional kind of economist and I'm a lapsed economist and we economists tend to think about how people make decisions and try to understand how they part with their hard earned money, how they spend their money. You started with monkeys at Yale? Tell me a bit about that.
Keith: When I was in grad school, really interesting and powerful finding that came out that shaped a lot of the way I thought about things. So when I was graduate school, learning all of these kind of fascinating findings in behavioural economics I thought to myself well something else that's really striking is how universal they see. This is not just a British way of thinking about money or a Chinese way of thinking about money or an American way of thinking about money. This just seems to be deeply human. And the question was will does that teach us that it's some fundamental feature of our cognition. But that's a little hard to establish because you could imagine that in some sense globalisation means we're all kind of subjected to the same basic market forces and those market forces are pretty common across a lot of different settings.
So one thing that I was curious about as well, is it possible to think about how universal our kind of hangups and our behaviour is towards money by asking a broader question which is saying well one way of establishing that the ways that we think about money are universal across all humans would be to say well it's even broader than that it's universal across a wide swath of primates.
Sandra: What Keith was trying to gauge was how far along the evolutionary chain did the association of money as a measure of value hold true?
Keith: And so actually, you know, without much of a clue or of a sense of well-founded hope that this was going to lead to anything, some colleagues and I began plotting to teach two groups of monkeys how to use money.
Sandra: Keith set out to challenge the belief that humankind's knack for monetary exchange belonged to us alone. Monkeys have a bottomless appetite for sweet foods - so using marshmallows, apples and grapes as rewards, Keith and his collaborators proved that monkeys can learn to use coins - hard inedible metal- as something of tradable value. The monkeys not only understood they could use money to buy delicious food, they also learnt to use money to conduct more complex transactions such as taking a gamble. In his experiments the monkeys learn to express their preferences for risk in that they might be willing to forgo immediate enjoyment of their treat and subject themselves to the possibility of a loss or gain just on the toss of a coin. The monkeys even displayed human like reactions to unexpected events like price shock when grapes suddenly cost two coins they would buy less and price discounts - the apples are half price, let's buy a few more!
Keith: Interestingly though when we started to investigate whether or not these groups of monkeys when put in very very similar situations to humans in economic decision making settings, what was amazing was that there was no statistical test we could run that on some very very basic levels could establish a difference between these monkeys and the median American stock market investor.
Sandra: Keith and his colleagues decided to test how the monkeys responded when they were deprived of something they expected, or hoped, to get - compared to how they felt when they received an unexpected treat or benefit. In economics this is the concept of loss aversion - the apparently illogical psychological response that says we humans feel more pain at losing something than we feel joy when gaining something of equal value. For example...
Keith: How upset do I feel when something that I planned to purchase for 10 dollars now cost 12 - subjecting me to an unexpected loss of two dollars. How upset does that make me feel vs. how happy do I feel when something that I plan to buy for 10 dollars is now on sale for 8 dollars and subjecting me to an unexpected $2 gain?
Sandra: Study after study has shown that psychologically humans feel the pain of loss twice as powerfully as the pleasure of gain. Keith wanted to know how far along the evolutionary tree this loss aversion travelled.
Keith: This appears to continue up until the point when it just doesn't. Like pigeons on this dimension are much smarter than us. They weigh that gain and that loss equally, it is just two dollars. You should feel just as sad losing two dollars as you feel happy finding two dollars and even some very distant primates like lemurs appear not to show any kind of asymmetry. What's kind of cool about those results is it kind of almost allows us to in some sense pinpoint within 10 million year range exactly when this kind of way of thinking about gains and losses, this kind of loss averse part of our psychology appears to kind of enter our cognitive toolbox.
Sandra: So does culture amplify that?
Keith: It's a little bit hard to put a number on how much culture amplifies vs. just kind of reflects this innate tendency but one thing that we do see in some behavioural economics studies is that people when they get a lot of regular feedback can train themselves not to react in irrational ways to these gains and losses. Professional poker players you hear a lot about teaching themselves not to steam. They just took a loss, that's no reason to lose your head and then make a stupid bet on the next hand, and good professional poker players teach themselves not to feel that way. Stock traders with some large amount of experience kind of show this. John List, a prominent cognitive behavioural economist found that professional baseball card traders, because it turns out he was interested in baseball card trading, don't show this after a good amount of experience. What's interesting is that even those professionals who have learned to show this in their professional lives, when you take them just 10 feet away from what they're good at immediately start sharing this again. So you know the stock market trader if his hobby is to trade baseball cards even though he's trained himself not to behave in this particular way with his day job when he's trading stocks will immediately start behaving this way when someone offers him a less good deal than he expected on his vintage Babe Ruth and vice versa the professional baseball card trader who is really really dispassionate and smart about that did some really crazy things in his retirement portfolio.
So what's interesting is that it paints this picture of this very innate, ancient parts of our psychology that of course we can overcome with some thought. That's part of being a self reflective human but that's still deeply a part of us that we kind of revert to quite naturally and easily. You've studied capuchin monkeys and how they behave when they get unexpected amounts of food with regard to how much money they've decided to pay that food. How have you carried over some of those insights that you've gained there in your role at Uber and what is your role at Uber?
Keith: I should say that this is in the past it was my role at Uber recently I've returned full-time to UCLA as a professor of behavioural economics, when I was at Uber one of the main things that my team worked on and thought a lot about was how to get both drivers and riders on the Uber platform to act dispassionately and for drivers make more money and for riders that take the most convenient ride for them. It's interesting because we often think about people as needing kind of nudges to work and keep good work habits. Let's take drivers for instance so the core of the business model was that Uber only makes money when the drivers make money. So Uber roughly speaking takes 20 percent commission of all of the trips that riders book.
So Uber as a platform only really makes money when drivers make money and in that dimension their interests were very well aligned with drivers and a lot of what my team at Uber tried to do was to help drivers make as much money as possible in as short a time as possible and maximise their hourly earnings. And in doing so help drivers appreciate the platform hopefully spend more time on the platform and make both themselves and Uber more money. That was something that was really important. And you could imagine a couple of dimensions on which you could do that. So one dimension is trying to help drivers figure out if they could preplan being on the platform what times of day would be most useful for them. A more important thing would be if there are times when the platform really needs more drivers like rush hour, a lot of people are using Uber to get around downtown. If you could give drivers a little bit of extra incentive to spend time on the platform at those times that was going to be a pure win for both drivers and for the platforms.
Sandra: Keith started to experiment using the nudge mechanism of surge pricing to see how it might change the Uber driver behaviour - Uber drivers being independent agents who are under no contractual obligation to roster on.
Keith: So early on surge pricing came into the picture as an idea where when there is an imbalance between the number of people who need rides, and the number of people who would be willing to provide those rides just a flat fare. We could increase the price to say listen hey now is a time when it would be really valuable to people trying to get where they need to go to have you on the platform. If you can spare a little bit of extra time you should you could imagine the presence of surge pricing doing kind of three things.
So imagine that the price goes up at some point in time in your city. You could imagine that it brings more people onto the platform. There's somebody sitting at home they're watching the basketball game and you know their team's losing and it's just getting depressing. If for example the little notification could pop up on the phone and say hey you know somebody just two blocks away from you wants a ride somewhere and how would you feel about taking them to the mall and earning 20 dollars. If this happens to be a time when you're losing interest in the game that might be a great idea. So it could bring new people onto the platform because we've just increased prices at a time when they're willing to work for that price. That effect turned out to be relatively small when people are not planning on driving on the platform.
Sandra: The price nudge touches Uber drivers in different ways depending on their current location.
Keith: You know it takes a lot to get them to change their plans and hop on. What turns out to be much bigger effects and what surge pricing was much more effective at was if someone's already driving on the platform they're already taking a few Uber trips, let's say this is a person who normally does three hour shifts. If it's surging, a very large observed effect was that drivers were willing to take a couple of extra rides at the end. Hey if we're getting paid twice as much as we normally do like the surge is 2.0 a driver who would normally do a three hour shift is more than happy to do a five hour shift.
It's really valuable because those extra two hours mean that the next day that they were planning to do another three hour shift they could take that entire day off and that turned out to be a pretty big effect. What surge pricing on a citywide level was able to do was not necessarily at any given moment in time bring more drivers online but it was able to give them extra incentive to the drivers who are currently driving to do a few extra trips. It turns out a really big part of surge was also the ability to get drivers both through the price mechanism but also because it gave them a good visualisation to move a little bit to the parts of the city where they were needed most.
Sandra: To appreciate the Uber story it's helpful to consider a few facts. It started nine years ago in San Francisco. Uber now operates in 81 countries and more than 600 cities. More than two million Uber drivers have carried out over five billion rides and by some estimates Uber is now worth around 70 billion dollars. And at the end of 2017 it was operating at a loss of about 4.5 billion dollars. One of the operating principles driving Uber's thinking was the service has to be....
Keith: As reliable as running water. But in order to do that sometimes there are more people who want rides than there are people who are willing to provide them or vice versa. So the search mechanism for riders the thing that we always hope that it would do would be allow people who need to go now to pay a little bit of extra to purchase that reliability and when you're late for a flight you can always get an Uber car.
It's just that if it happens to be at a time when a lot of other people need those cars too it's going to cost you a little bit of extra like 30% more or something like that.
Sandra: Uber customers also responded differently to price nudges and again Keith found that riders behaviours defied traditional economics.
Keith: One really interesting behaviour that we saw was that people responded very differently to price increases in that surge multiplier depending upon the exact number that was framed. So think about it this way, 1.0 surge that's exactly the normal price, 1.2 you're paying 20 percent more, 1.3 you're paying 30 percent more. And just like rational economics would suggest 1.2, 1.3, 1.4, 1.5 demand just kind of drops. A lot of people say to themselves wow I've had experience with this platform, it's 1.4 right now. I don't need to go right now, I can wait 15 minutes and see if the price drops and often it does within the next 15 minutes.
Sandra: But in real life it's not just about the money.
Keith: This does start to break down or what you start to see is interesting psychological phenomena. So 1.9, and that's a really rare event, Uber almost never gets to 1.9 but say a concert is getting out at a stadium near you there's just not enough cars to take everybody home.
The interesting thing is that between 1.9 and 2.0 there's a very big drop off. The amount that demand drops off between 1.9 and 2.0 is six times larger than the amount that demand drops off between 1.8 and 1.9 even though the absolute price change is exactly the same. What's also interesting is that at least for new Uber riders you actually see something that's even more puzzling from a normative economic perspective when the price goes up even a little bit more from 2.0 to 2.1. For new Uber riders slightly more people are willing to take rides at 2.1 than they are 2.0 even though at 2.1 those rides are slightly more expensive. And what appears to be the case is that imagine yourself taking a ride you're preparing to go somewhere and a warning flashes up and says hey listen you know we're really sorry demand is off the charts this ride is going to cost two times more than normal. Do you accept or do you reject vs. this ride is going to cost 2.1 times more than normal do you accept or do you reject. What's interesting is that what the evidence seems to suggest is that at least for new Uber riders that 2.1 seems not arbitrary. There must be a very fancy algorithm going on here 2.1 okay well that must be the fair price, fine I'll pay 2.1 whereas 2.0 they're just they're just ripping me off. Rides are twice as expensive right now, I'm not doing that, what do you take me for? And that's quite robust.
Keith: So a lot of what my team at Uber had to deal with were these interesting quirks of psychology that meant that the platform performed a little bit differently than the perfectly rational, highly tuned model of dispassionate economic agents would tell you. One initial proposal was well we think people should actually dislike higher prices and like lower prices and so if they're actually taking more trips at 2.1 than at 2.0 then we think they're probably making a mistake and we should take some blame for that. You know we're presenting this information in a way that is eliciting this response. One suggestion was well if this is how people treat these prices, the fully efficient thing that saves people as much money as possible while allowing as many trips to happen on the platform as possible would be to avoid surging 2.0 at all costs. As the search mechanism it says we should be charging higher prices to keep the service reliable you could imagine us holding surge 1.9 longer than we normally might have and then at some point jumping straight to 2.1 instead of 2.0. You could think of that as using behavioural insights to basically make decisions for new Uber riders as if they were experienced Uber riders. Help get a little bit more quickly to behaviour that we would see from experienced riders on the Uber platform. In the end we decided that even if that might have made sense, what deeper lesson this was teaching us was that this form of presenting the surge price information was not helping people make the best decisions in their own self-interest and the right solution was just to make the price more transparent. So what we started to do was instead of saying this is 1.3 times the normal price 1.5 times the normal price, we just started estimating that price for you and calculating it and saying oh you want to go to that museum downtown. The trip is going to cost you $10.67. Oh I'm sorry demand is off the charts, there are no cars near you. Now the trip is going to cost you $13.62. How do you feel about that? And when we started presenting prices in a much more natural way which you know to tell you the truth we should have been doing from the beginning but in terms of the dollar amount that it's going to take you to get from A to B you saw all of these kinds of somewhat irrational behaviours just immediately disappear.
Sandra: It sounds like you time at Uber has allowed you to gain some incredible insights and some of this is because of the tremendous data that Uber has and it's allowed you to think a bit differently about some of the types of problems that you could be investigating. Where to next?
Keith: Wow. I think as an economist who's had the good fortune to work with this amazing amount of data and really insightful data about people's behaviour, Uber and Uber's data have provided researchers insights into two what I think of as a really important changes in the way that we work, in the way that we live. So the first is that the possibility that technology like Uber, but I think this is only expanding to other areas of the economy, provide more and more flexibility to people and the use of their time and how they spend it and how they work. So as a professor I'm actually in one of the most flexible professions around.
I mean you know there's times when I have to be in the classroom and I have to be teaching and those are pre-scheduled. But besides that basically nobody tells me when I should be working, when I shouldn't be, where I should be working, I can work from my office or I can think as I go for a walk in the park, I have a lot of flexibility in the control and the use of my own time. What I had started to realise was that that's a tremendous luxury that I shouldn't take for granted. Most people who work in the economy if anything experience not just less flexibility but in fact negative flexibility. So for example if you work in the restaurant industry not only do you sometimes not have control over when you work sometimes you can get a call from your boss that says I'm sorry you know somebody else is sick and didn't come in for the chef, you're going to have to come in today. So that's like not only a lack of flexibility it's not only that you don't control your hours, you in fact don't even control the surprises and the surprises can be negative surprises. It's kind of negative flexibility. What I think is interesting is that technology is allowing more and more of the economy to move in the direction that kind of professors enjoy.
We're just going to have a lot more control of our time and the way that people respond to that is really fascinating. Uber and other kind of gig economy platforms are basically the first time in history that large swaths of the economy can basically tap their smartphone and turn on work and then whenever they want to take out their smartphone tap it again and turn off work. That's a tremendous change and I think something that is potentially revolutionary and that we don't fully understand the implications of yet but the Uber data gives us a first peek at what I think of as relatively massive change in how we get to organise our own lives.
Sandra: There will be unintended consequences of even things like flexibility for instance as much as Uber allows for more flexible work, it also has the ability to incentivise me to work maybe more than it's optimal for me or to get me to work when I shouldn't be working simply because of the incentives that it can build into the platform.
Keith: Yes certainly in a traditional kind of world where your job asks you to be there from 9:00 to 5:00. In an ideal world just giving you complete control over those hours can only make you better off. It just gives you flexibility to kind of make your work kind of work for you as opposed to the other way around. But certainly you could imagine a transitional period where people have to learn how to deal with that flexibility. And we all have experiences where sometimes a little bit of rigidity kind of helps us. We can set up arbitrary rules for ourselves about every morning having to go to the gym between six and eight am and that rule kind of maybe not being exactly the right thing in the middle of a snow storm or in the middle of a heavy rain, but we just kind of feel compelled to stick to it because that kind of rigidity actually helps us all live our lives a little bit better.
Keith: I get the feeling that in the advent of technologically provided flexibility in how we all work we're all going to have to become a little bit more sophisticated about the rules that we impose on ourselves in order to make that new world just work a little bit better for us.
Sandra: Beyond Uber lies the yet to be realised era of self-driving vehicles. Keith thinks autonomous vehicles are coming and their impact will be profound.
Keith: In both a really liberating way and potentially a really disruptive way.
Sandra: Up to now professional drivers have been immune to the off-shoring encountered in other low skilled jobs. Not only might autonomous vehicles decimate these jobs the impact could be huge, not in the least because...
Keith:...long haul truck driving is over 3 percent of the American workforce which is a surprisingly high number. And in more than 20 states in the United States it's the most common job that people who live in that state report. So I think that's going to be extremely disruptive, but hopefully more efficient and safer for the economy. It also turns out to be a relatively taxing job both on people's personal relationships and their health, as a whole a somewhat dangerous job.
Sandra: Keith also predicts that driverless cars will change the way we work and the shape of our cities.
Keith: A relatively persistent pattern in the United States has been that the average commute for an American is remarkably constant across time at around 37 minutes. You build a brand new highway that on average increases commuting speeds from 50 miles an hour to 65 miles an hour.
People move just a little bit further out to that next beautiful suburb that's just been built and basically have no change in their commuting time. I think self driving technology may be the first major technological change that kind of cracks that nut. I think I would be willing to commute an hour in the morning if what I'm doing during that commute is not having to pay attention to the road and drive but instead could kind of sit back, check my email, check my Twitter feed. It's a little bit more like allowing all of us to enjoy a train commute as opposed to driving. And I think it's got to make car travel dramatically more efficient - when cars are self driving, it's possible for one lane where all of the cars in that lane are self driving and can be speeding along at 80 miles an hour only a foot between each car just bumper to bumper. That's both much more efficient because chained cars like that save a lot of energy because of minimising air resistance but also that means that that lane can both experience zero traffic setbacks and also just carry a lot more people. And I think that efficiency is going to mean a lot for people's lives.
Sandra: Is there anything you're afraid of?
Keith: Do you think that even a techno optimist has to pause and say that with the advent of new technology at the very least users we're all going to have to grow up a little bit in our use of technology in understanding its applications for how we live. So we don't quite understand the extent of it yet but the degree to which social media and what is called like fake news spreading on social media had an impact on the last US national election I think, it is not fully understood but appears likely enough that we kind of really have to understand how does social media change the way that we all participate as citizens in a democracy. I do think that we're going to all have to become much much more self-conscious protectors of our own privacy and stewards of our own data in ways that we never have been before. So in principle you could always have learned a lot about a person by sifting through their garbage every week. But we were all kind of protected from that a little bit just by the fact that it's kind of inconvenient to sift through somebody's garbage. And maybe I've been lucky but I've never been interesting enough of a person to ever have someone want to sift through my garbage. But now thanks to technology sifting through millions of people's effectively kind of digital garbage, using machine learning techniques and using kind of big data techniques, has dramatically dropped that barrier. Because of that, things that we didn't need to use to worry about we may have to become all a little bit more self-conscious about.
Sandra: Keith, thank you so much for talking to us today.
Keith: Yeah thank you so much I've really enjoyed it.
Sandra: If you'd like to hear a bit more from Keith on his groundbreaking work with money or monkeys, you should definitely check out the University of Sydney Business School's Global Executive MBA program. In the meantime here's a short clip from our fellow podcaster Stephen Dubner from Freakonomics. Dubner is describing one of Keith's more surprising findings, when he was working with the Yale Capuchin monkeys, a discovery he did not intend to make.
Audio from Freakonomics: And then out of the corner of his eye, Keith Chen would see something that later he would really regret having seen which is one monkey who is still holding on to a coin. He goes over to the second monkey and gives the second monkey the coin and now Keith Chen the economist is thinking what am I seeing in the monkey economy - is it monkey altruism? Is it the repayment of a loan of some kind? There were a couple of seconds of grooming between the monkeys and then yeah bam it's monkey sex. It is the first recorded instance of monkey prostitution in the history of science. And then to show how thoroughly the monkeys understood the concept of money by now after the sex was over which was just like 8 seconds because they're monkeys - after the sex is over the monkey who had received the coin for sex probably marches it over to Keith Chen to buy some grapes with it.
Sandra: People like Keith Chen are part of the group of international thought leaders involved with the University of Sydney Business School's Global Executive MBA program. To find out more our immersive, experiential learning delivered in multiple countries around the world, from the UK to India, from Israel to the US go to our website mba.sydney.edu.au.
Sandra: This podcast was made possible by Jacquelyn Hole and Megan Wedge who made this story feel good and sound awesome.
Outro: You've been listening to Sydney Business Insights, the University of Sydney Business School podcast about the future of business. You can subscribe to our podcast on iTunes, Stitcher, Libsyn, Spotify, SoundCloud or wherever you get your podcasts. You can visit us at sbi.sydney.edu.au and hear our entire podcast archive, read articles and watch video content that explore the future of business.
Close transcript