Sandra Peter and Kai Riemer
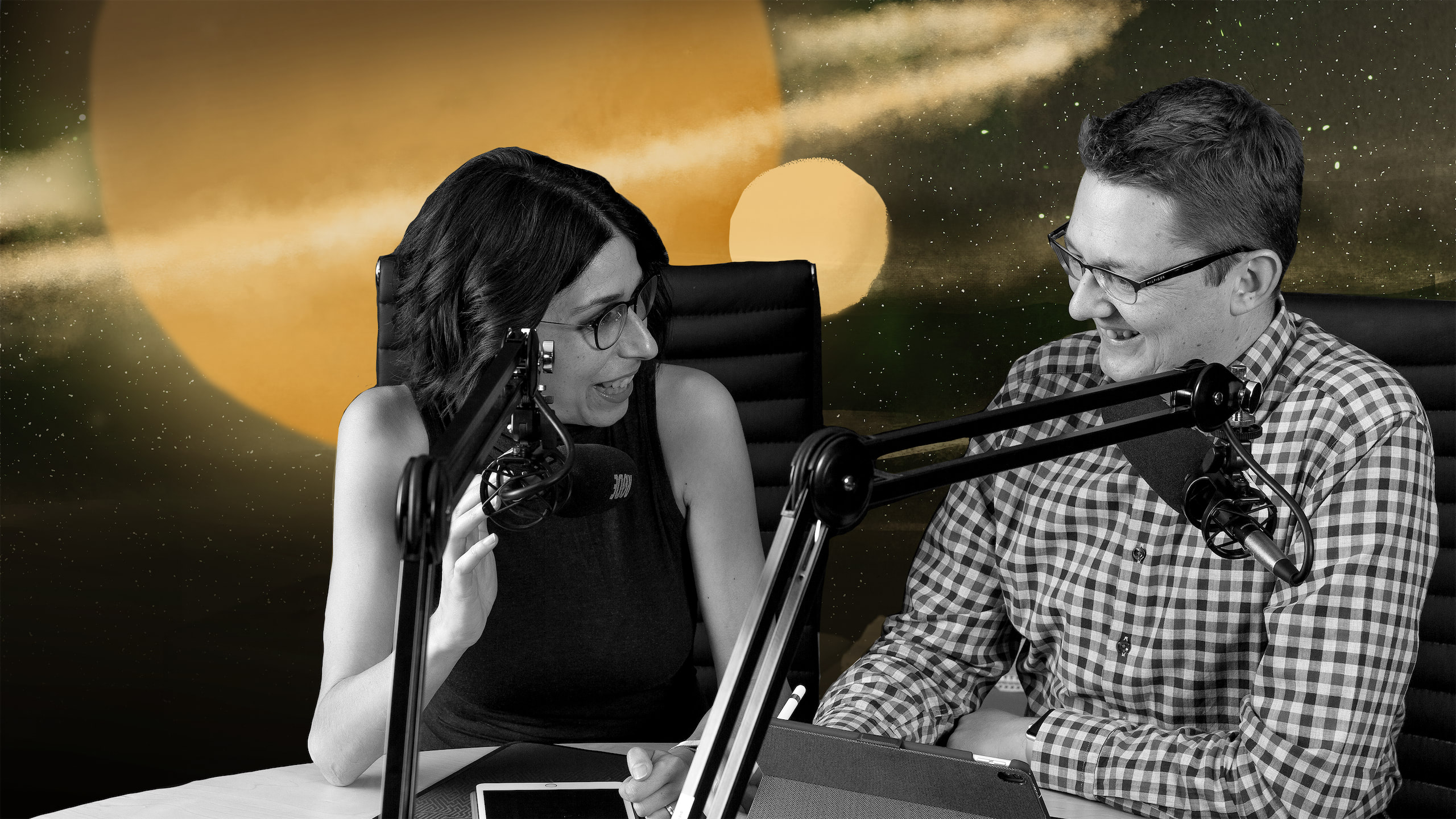
The Future, This Week 27 October 2017
This week: scary tales about superhuman robots, a global waste blockage, and tricking cars. Sandra Peter (Sydney Business Insights) and Kai Riemer (Digital Disruption Research Group) meet once a week to put their own spin on news that is impacting the future of business in The Future, This Week.
The stories this week
Google’s AI ‘no longer constrained by limits of human knowledge’
The Chinese blockage in the global waste disposal system
Other stories we bring up
Artificial intelligence: learning to play Go from scratch paper in Nature
DeepMind’s Go-playing AI doesn’t need human help
What will happen to the world’s recycling
China tries to keep foreign rubbish out
Antenna Documentary Film Festival
Historian Josh Goldstein on China’s economy and the international recycling trade
The Future, This Week 24 March 2017
Robot of the week
Join us together with AmCham at our research breakfast series on:
The future of health: reimagining healthcare
You can subscribe to this podcast on iTunes, Spotify, Soundcloud, Stitcher, Libsyn or wherever you get your podcasts. You can follow us online on Flipboard, Twitter, or sbi.sydney.edu.au.
Our theme music was composed and played by Linsey Pollak.
Thank you to Ross Bugden for “♩♫ Scary Horror Music ♪♬ – Haunted (Copyright and Royalty Free)” which is featured in this podcast.
Send us your news ideas to sbi@sydney.edu.au.
For more episodes of The Future, This Week see our playlists.
Dr Sandra Peter is the Director of Sydney Executive Plus at the University of Sydney Business School. Her research and practice focuses on engaging with the future in productive ways, and the impact of emerging technologies on business and society.
Kai Riemer is Professor of Information Technology and Organisation, and Director of Sydney Executive Plus at the University of Sydney Business School. Kai's research interest is in Disruptive Technologies, Enterprise Social Media, Virtual Work, Collaborative Technologies and the Philosophy of Technology.
Share
We believe in open and honest access to knowledge. We use a Creative Commons Attribution NoDerivatives licence for our articles and podcasts, so you can republish them for free, online or in print.
Transcript
Introduction: This is The Future, This Week on Sydney Business Insights. I'm Sandra Peter. And I'm Kai Riemer. And every week we get together and look how the news of the week. We discuss technology, the future of business, the weird and the wonderful and things that change the world. OK let's roll.
Sandra Into: Today on The Future, This Week: scary tales about superhuman robots, a global waste blockage, and tricking cars. I'm Sandra Peter, I'm the Director of Sydney Business Insights.
Kai: I'm Kai Riemer, professor at the Business School and leader of the Digital Disruption Research Group. So Sandra what happened in the future this week?
Sandra: Scary news this week from Fox News.
Kai: Aren't they all.
Sandra: Yes but this is particularly scary on this scary week. This one's about robots. Google's artificial intelligence computer is no longer constrained by human knowledge. It comes complete with a picture of the Terminator.
Kai: So this is a story about AlphaGo the artificial intelligence software program built by Google subsidiary DeepMind that has previously and famously beaten the world's best human players at the board game Go which is the ancient Chinese boardgame widely known as the most complex and difficult board game known to human kind. Previously AlphaGo was able to beat the best human players. What is new now is that AlphaGo Zero, the latest machine learning program, has famously beaten all prior instances of AlphaGo and it has done so in a very different way. So what this team has done is they have employed a different machine learning algorithm which does not learn from prior human games or prior human knowledge but has figured out how to play the game all by itself.
Sandra: So here is David Silver talking about this, and David Silver is AlphaGo's lead researcher, the first of the 17 researchers who together wrote the "Mastering the game of Go without human knowledge" - article that appeared in Nature last week and we'll include a link in the show notes.
Audio: AlphaGo Zero which is learned completely from scratch, from first principles without using any human data and has achieved the highest level of performance overall. The most important idea in AlphaGo Zero is that it learns completely tabula rasa, that means it starts completely from a blank slate and figures out for itself only from self play and without any human knowledge, without any human data, without any human examples or features or intervention from humans it discovers how to play the game of Go completely from first principles.
Kai: So Sandra what David describes here is a different form of machine learning that works unlike the kind of algorithms that we have discussed on The Future, This Week previously. So let's recap how traditional machine learning works.
Sandra: So we spoke previously on the show about two different types of machine learning. The first instance, 50s 60s 70s, was when we tried to encode all of our knowledge into the algorithm. So what we tried to do is if we wanted the algorithm to recognise a picture of let's say a cat we tried to describe that cat in every single possible detail and encode that in the machine. Of course this ran into some problems because of our limits to explaining everything that we see in the world around us - there are cats without fur, there are cats that are hideously ugly, there are cats that are hiding in the bushes and so on. And we didn't get very far with this way of encoding knowledge.
Kai: So that's what we commonly call good old fashioned AI. It's basically a way of encoding explicit knowledge about the world into the algorithm and it's strictly facts and rule based. But we all know that this is not how the human world works and when this program encountered the common sense problem it basically broke down and it sent AI into a kind of an ice age from which it has recently awoken because we discovered the power of neural networks and machine learning.
Sandra: And also our computing power got a lot better and the datasets that we had and we could use got a lot better. So instead of our previous approach we had an approach called 'supervised learning' in which we told the computer here is a million pictures of cats. You go and figure out what a cat is and we told it this is a picture of a cat, this is not the picture of a cat. And eventually the computer got pretty good at recognising cats in pictures.
Kai: And the same you can apply to the game Go. So the first instance of AlphaGo was trained with more than 100,000 games that were previously played by humans. And so it learned about the strategies that humans would employ and it learned to become a really good player. It learned to improve on those strategies and then eventually it beat the best human players in the world.
Sandra: But we still run into the problem that in order to train the first instances of AlphaGo we needed to actually feed it tens of thousands, 100000 human games of Go and human strategies and the way that we have figured out the game can be played. So now comes AlphaGo Zero.
Kai: So AlphaGo Zero is based on what is called reinforcement learning. It is still built on the basis of neural networks which you could call them statistical weighting machines. They're basically networks of hundreds of thousands of millions of these statistical values that are basically adjusted to recognise certain patterns. In this instance patterns of game play. What is novel here is that AlphaGo Zero learned to play the game as David said from first principles. So what the algorithm was given is the rules of the game. At any moment in time, the current state of the game, so full information of what the board is like and then crucially a function of what each particular move that it was now able to make would do for its chances of winning the game.
Sandra: And it obviously knew what winning the game meant.
Kai: Absolutely so there's a lost function against which the algorithm is then able to optimise. So what they then did is they let this algorithm play the game against itself. Four point nine million of those games over three days, in which AlphaGo Zero basically learned by trial and error those strategies that were more likely to let it win the game and because it wasn't trained with prior human games it was able to come up with moves that no human had ever played that were completely new to the community of Go players. And it was therefore able to figure out how to win and how to become the best entity at playing Go all by itself.
Sandra: So why are people so scared the about this? Why is Fox News and other channels reporting that we finally built super human AI? Why are there pictures of the Terminator? Did this algorithm really learn without any human intervention? And if we go back to David Silver's explanation, he emphasised a lot that there was no human knowledge, no human expertise, no human intervention in this. Is that really true?
Kai: Well yes and no. So first of all if you look at the world of AI then it is significant that the algorithm was able to achieve this without human data, so previous data of games, and if you only take this message and you create a headline and you put it into people's Facebook feeds. It sounds scary right? So there's an algorithm supposedly learned to play AlphaGo all by itself. So imagine what this thing could do if you let it loose on the world. Right. But that's not how it works. So this algorithm was given quite a lot really.
Sandra: It was given a very clear problem the game of Go. It was given the very clear rules, what is happening through the game of Go. It was given all the information it needed - what the board looks like at every given time - and it was given a very clear unambiguous outcome, win or lose situation.
Kai: Yes. So it was able to actually optimise against a very clear set of criteria and then what was done was a brute force approach to play four point nine million games by which the network was then able to learn the patterns to achieve the intended outcome of winning every time.
Sandra: So still quite a bit of brute force and definitely an advancement over previous versions of AI. It used a lot less computing capacity to achieve this.
Kai: So instead of 48 processor cores as in the previous AlphaGo version, the team was able to come up with an elegant algorithmic solution that only needs 4 processor cores and that's significant because you can build that into one machine, you can have one computer that you can actually carry around and supposedly in a short period of time you can run this on a laptop computer. You don't actually need a data centre of 12 computers running with a lot of processor power using a lot of energy. So there's a lot of advances in the elegance of the algorithm and in the computing power that is needed.
Sandra: So the computer only played against itself for 72 hours before it was able to defeat AlphaGo but it's still played 4.9 million games. That is still quite a bit of training that you need to do to achieve this. So Kai why are we still so scared then about this? Why is this still so scary? Is it just because it's the week before Halloween?
Kai: I think the reason is the way in which we think about and talk about these algorithms. We talk about intelligence, we talk about learning, we talk about the algorithm figuring things out without the help of humans. So we're ascribing a kind of agency to these algorithms that suggest that there is something intelligent there that you know they give a shit about what they do. And then we say imagine where they could go you know if you put them into the world and they figure out problems around us and then they learn more and more about our world and they develop this intelligence and then eventually they surpass our intelligence. And of course all of that is bullshit. And if you read on and even in the Fox News article right if you read it to the very end, things become much much less scary.
Sandra: The end of the Fox News article actually reports on Satinder Singh who is from the University of Michigan who wrote a commentary that was originally carried by Nature as well, that said that this is really not the beginning of the end because AlphaGo Zero like all others successful AI so far is extremely limited in what it knows and what it can do compared with humans and even other animals.
So let's think about this a little bit because the types of problems that the algorithm would encounter in a game like Go the one with clear rules and clear problems and clear outcomes, perfect information. There are not many problems in our real day to day life that subscribe to that. Quite often what we want to achieve is ambiguous, the information that we have about the state of the world is ambiguous and the rules of how to go about doing this cannot easily being coded or sometimes not even known.
Kai: Yes so most decisions in life especially those that matter are happening when we live our lives forward. We do not know what an optimal outcome would be. There is no mathematical function that we can optimise against. We do not have perfect information so the idea that we can just take reinforcement learning as in the AlphaGo Zero example and apply it to many other areas of daily life, it's just not possible. So that's a generalisation fallacy or an extrapolation fallacy which basically captures the idea that "oh look at what we have achieved now in such a short amount of time. Imagine what this thing can do once you put it on to other problems." The problem is that those other problems are of a different kind, right, so you cannot just transplant this algorithm and put it on any old problem. However there are areas of application where this can be potentially immensely useful especially in the sciences.
Sandra: So an example of that is actually given in a Verge article, which we'll include in the show notes, by DeepMind's co-founder Demis Hassabis and he talks about the fact that for instance Alpha Zero could eventually be used to search for a room temperature superconductor. This is a hypothetical substance that would allow electrical currents to flow with zero energy loss allowing for incredibly efficient power systems. Now we do have these superconductors already so we know how this works. But they only currently work at extremely low temperatures so employing something like AlphaGo in this sort of domain might provide some real advances for what we can do now.
Kai: So these are areas where we know what we're looking for, we know what we want to optimise, it's reasonably bounded space in which we're searching much like a game which has a board which limits the space in which things happen. It also only has a bounded amount of elements in play which in this instance would be different materials. So once we have all of these conditions we still have the problem often times that the number of combinations is incredibly large and very complex. So Go, for example, the number of possible combinations that you can play in Go exceeds the number of atoms in the universe. That's significant right. So we're talking these kind of complexity problems which you find in certain areas of the sciences so potentially this algorithm can help us figure out certain of those combinatorial problems that we wouldn't be able to solve by other means. But that does by no means mean that we're any closer to solving the problem of creating a human kind of intelligence in machines.
Sandra: No. And we will never give this machine the rules of physics and the machine would come up with a new domain of physics we should be investigating. Nor is this machine going to find hidden variables that we never considered from a different domain nor will it rely on chance or luck to achieve certain outcomes. Most important of all I think we have not come any closer to achieving anything that gives a damn about what it does. The first generation of AI that we spoke about didn't care what a cat was nor did the second generation of AI care what a cat was. Nor does AlphaGo Zero care about maybe playing chess or not playing anything at all.
Kai: And that's why we fundamentally disagree with the last statement in the Verge article which says these caveats aside the research published to date does get DeepMind just a little bit closer to solving the first part of its tongue in cheek two part mission statement - part 1 solve intelligence, part 2 - use it to make the world a better place. So the answer to that is no. This does not get us closer to solving intelligence. It might get us closer to understanding better what intelligence actually is or isn't. But I think it's a false assumption to make that if we just keep improving the algorithms we have today we will eventually build artificial general intelligence. There is no evidence for that. It's likely that this is a problem of a very different kind that we have no idea today how to solve. That does not take away anything from the success that these scientists have achieved because we are now able to create new tools that will probably allow us to solve a new class of problems. But calling it artificial intelligence and scaring people does not help anyone.
Sandra: As for making the world a better place, as you know I am generally a techno optimist and I think technology in general is bringing us great advancements and I'm in love with every new gadget that comes out. But as much as you can use these algorithms to solve the world's problems you can also use them to amplify some of the world's problems or to take advantage of people or to game certain systems. So hopefully it's a small step towards making the world a better place.
Kai: So this is not such a scary story after all despite the Terminator picture in the Fox News article but our second story is quite the opposite, it starts out as a more or less boring innocent story but turns out to be quite the scary one.
Sandra: This is a truly fitting story for the week just before Halloween.
Kai: Yeah this one is truly scary. It's from the BBC. It's called the "Chinese blockage in the global waste disposal system".
The story talks about the global waste disposal system. Three months ago on July 18th China decided to ban twenty four different types of rubbish as part of its "National Sword" campaign and this campaign was against foreign garbage. Turns out that China actually plays a huge role in the world garbage disposal system.
Kai: This article is interesting because it gives us some facts that at least Sandra and I didn't quite know to this extent. Apparently countries like the US, Australia, many European countries, export much of their waste especially the kind of materials that are collected as part of the recycling system and then export these materials to other countries of which China is one of the largest recipients.
Sandra: China has actually been the largest importer of many types of recyclable materials and a good article in the conversation talking about China banning foreign waste tells us that last year Chinese manufacturers have imported 7.3 million metric tons of waste plastics from developed countries so countries like the UK or the EU or the US, Japan and even Australia. So the stance that China took back in July and notifying the World Trade Organisation that it will ban these 24 categories of recyclable material was to say no to foreign garbage and this applies across the board to plastics, to textiles, to mixed paper which means that China will indeed take a much smaller role in recycling the world's garbage.
Kai: So there's a combination of problems here. One is that a lot of waste that is exported from countries such as Australia, they're simply not clean. This might be companies that are in charge of recycling cutting corners so the plastics and paper waste and other waste that is exported might be contaminated with things that then create problems. But it's also certain materials that China is just not prepared to take any longer. So the submission to the WTO reads "We found that larger amounts of dirty wastes or even hazardous wastes are mixed in the solid waste that can be used as raw materials. This polluted China's environment seriously." So what China is doing is taking a stand for its citizens and says certain types of materials are just no longer acceptable to be taking into China because it creates such problems for our environment and the people who are dealing with these wastes. And we found some really scary and sobering information online about the types of jobs that are attached to this industry in China and the lived realities of the people who have to deal with this garbage.
Sandra: And we'll include in the show notes a link to a new documentary called Plastic China which was showed last Sunday in Sydney as part of the Antenna Documentary Film Festival. A very powerful documentary showing the lived realities of these people.
Kai: So Sandra for many of us garbage ends at the bin right? So we put our stuff into the red or yellow bin if we recycle the garbage truck comes and that's as far as we're concerned with what happens to this stuff. But how did we get into the situation that much of our recycling materials are going overseas and they're taken in by China. And what does it all mean?
Sandra: We got to where we are I think through a combination of a number of things. First and foremost is the fact that yes we do produce a lot of garbage. We do not recycle most of it in the developed countries and we are sending this garbage to a number of countries and China is not the only one. There are other places like Ghana and Nigeria and Pakistan and India that we're selling garbage to but we send off this garbage to be processed. Now China over the years has become the world's largest manufacturer. It's got a leading position in global manufacturing. And through this economic power shift, which is one of the megatrends that we look at here at the University of Sydney Business School, China has assumed the role of processing all these materials. Now this has to be seen in conjunction with increasing regulations both here in Australia and in places like the European Union around what kind of garbage we can put in our own landfills because we don't want to pollute our environment so we increase not only the waste that we produce but also we decrease the quality of the waste that we send over to China. So increasing regulation. And third let's not forget that China has a booming and growing middle class and this middle class produces increasing amounts of garbage itself. Kishi one of our Associate Lecturers at the business school yesterday actually was telling me some statistics around the fact that since last year the number of people who order online in China since last year has grown to such an extent that active users that is people who order food out more than three times a week is around 400 million in China. So a total number of customers who order food out is about 600 million people. This is a lot. Nearly half of the population ordering takeout that has a lot of plastic bags, plastic containers, that all end up having to be recycled in China.
Kai: So there's a combination effect now. China does not want certain materials from other countries any more but it is also now in a situation where it has huge amounts of waste that it has to recycle itself. Now let's not forget these plastics that China is importing they're not doing this out of you know the good of their heart to help other countries get rid of their waste. These are resources that they can use for their industries. So they're actually quite valuable.
Sandra: Surprisingly expensive resources. So a final bit of statistics for today coming from the U.S. China Institute at the University of Southern California: so expensive and so valuable is this garbage that back in 2004 the United States' export of crap basically, of scrap, to China was the biggest dollar value export to China. It outstripped everything else. Aeroplane parts, electronics everything else they imported a huge amount of goods from China. And their main export, their highest value export to China back in 2004 was trash.
Kai: Now this creates huge implications for Western countries who in the past just happily got rid of this waste by putting it on ships and sending in offshore who now have to basically figure out what to do with this stuff. Should the WTO Grant China's request to stop these imports?
Sandra: So where to now? Let's have a look at the impact because this ban has been on for three months now and garbage is starting to pile up in various countries that used to export this thing. So what is the impact of this?
Kai: So on the one hand Western countries might just say we're just looking for another place to put our stuff and other countries might pick up whatever China is not prepared to take. So that's the literally quick and dirty option. But maybe this looming crisis might actually set incentives to invest in new technologies and modern ways of recycling and garbage disposal in the countries of origin. Now we see that regulation for example can spur innovation in a lot of these industries as has been done in Europe. Germany for example is not only an exporter but also an importer of waste because in many places there are modern facilities for recycling and incinerating wastes and the BBC article also tells us about innovations by start up companies who are basically able to turn plastics for example back into oil based products which then become raw material to create new plastic. So maybe this is actually the kind of crisis that will spur out of necessity innovation in developed countries.
Sandra: But let's remember this is still a very scary story because the garbage is piling up as we speak and actually the investment required to build a new recycling plant can be quite large. The time it takes to get one up and running and to rethink supply chains and value chains takes actually quite a bit of time and money.
Kai: So this is not the kind of problem that you solve by just stopping the system, figuring out the problem and then restarting it. When we talk garbage recycling, garbage facilities, in developed countries we very quickly run into the "not in my backyard" problem right. So it starts from very simple problems like where do I put those facilities? What are the regulations? Environmental planning processes? So these things can take years and years to simply be built and that's before we have actually figured out what to do. Who is going to solve this problem.
Sandra: So there is a real risk that actually we might just move this problem from a place like China to other places that are maybe even less equipped to handle our waste. So places like Pakistan or Nigeria or Ghana other parts of Asia and Africa and move this problem down the line in another 10 years, another 20 years.
Kai: But it also reminds us that we actually have to start solving this problem at the source by simply creating less waste. And that starts in the supply chain by thinking about alternative ways of packaging using paper instead of plastics or simply by changing our own behaviours and not buying products that are heavily wrapped in plastic. So I think this is the kind of problem that cannot be solved by treating the symptoms which is once we have created waste but which will collectively require a rethinking.
Sandra: And let's not forget China who is in the middle of this discussion. The impact of this on China might actually be a good one.
Kai: China is doing this for the sake of its own citizens and it comes at a price because there is jobs and employment attached to those industries that will probably have to be reduced. But China has made the call and said there are certain things they are not worth doing and it's more important to take care of the environment in the face of rapid urbanisation especially, where China is producing more and more waste. It has to take care of its environment and we've seen that China's doing this across other industries like energy production as well. So they have been on a big campaign to improve the environmental reality in China. So this is just the latest move and it tells us in the West that we will less and less able to simply export our own problems.
So our last story for the day is a happier one. This is a tongue in cheek one where we're scaring cars by tricking them. So this is about self driving cars again.
Sandra: So this story is from Wired magazine titled "In New York self driving cars get ready to face the bullies".
Kai: So Wired reports on efforts by General Motors who is putting its self-driving Chevvy Bolts onto the streets of Lower Manhattan early next year.
Sandra: The company is already doing this in places like San Francisco and hopefully soon is about to learn from New Yorkers how people interact with autonomous vehicles.
Kai: So the author is speculating about how the quite aggressive nature of traffic and pedestrians in lower Manhattan.
Sandra: Eh I'm walking here.
Kai: That's right. Will actually interact with the self driving cars that are by their very nature conservative and rule abiding. And so the article paints the scenario that these poor cars will just have to stop every time a pedestrian steps into the street and you essentially get nowhere because they're not going to be able to really face up to the bullies who will trap them in the streets.
Sandra: And we can see this happening. We came down in an Uber to work today and there were lots of students on campus walking all around the car and in front of the car and next to the car and cutting in and bicycles cutting in on everybody else.
Kai: And we can't say that the driver cared too much for those people and was pushing on. So if we had been in a safe-driving car that will stop and be cautious.
Sandra: We'd still be there.
Kai: That's right. There would be no podcast this week.
Sandra: So this is about humans bullying cars.
Kai: This is about people figuring that they can play pranks on these cars which are designed to abide by the rules.
Sandra: We've seen this on the podcast previously. Right? We're talk about someone playing pranks on autonomous vehicles?
Kai: Yes and we've discussed this similar story but that was more speculating, now this is actually happening. And so companies are trying to deal with this in various ways. And at the heart of this problem lies the fact that human life does not proceed just by a set of rules. Humans are spinning intricate webs of norms and behaviours and interactions that we're really good at. We don't have to think about this. We can just cruise in traffic, we can just get along with you know sometimes we get upset and we give someone the hand or the finger. But by and large our human world works with but also outside of the rules. But a machine needs definite rules to actually on the one hand to have something to go by but also to make sure that the companies selling these self-driving cars don't get into trouble because their cars are actually breaking the rules.
Sandra: So therein lies an interesting problem because so far we can't make cars that are good enough at reading our facial expressions or really figuring out whether we're looking at the person on the road or we're looking at the traffic lights or we're looking at our phone, at whether we've just raised our hand or whether we've lifted our middle finger.
Kai: And in the absence of a human driver we appreciate these cars being conservative and just breaking once too often rather than too late.
Sandra: So then our only remaining solution seems to be to actually design more rules for people or redesign streets or redesign the way we interact with our built environment so that we actually keep people out of this. They're bothering the cars.
Kai: Yeah. So there's actually two sides to this story. So on the one hand people are trying to figure out ways to design into the cars ways of signalling pedestrians for example what the intention of the car is. One solution is projecting light onto the road in front of the car you know where the cars going to go. Or creating new kinds of indicators that are sitting on the roof of the car. So there's different solutions that seem a little bit awkward. And it might take a while for them to be established and catch on. But the other solution that is mentioned in the article is a bit concerning because it's about enforcing the rules that already exist, making humans adhere to the rules, changing the built environment so that it is more in line with what self driving cars need.
Sandra: So we must respect the self driving car.
Kai: Yeah. So in essence what we're saying is turn humans into more like machines so that machines can get on in our world.
Sandra: Are we losing track of why we're doing this in the first place?
Kai: Maybe so if we say that we want self driving cars because they're safer but if we then say that in order to have self driving cars in the street we need to better police the rules, build the environment such that it becomes safer for these cars to drive. Do we actually still need the self driving cars? Or can we not just create a safe environment by doing these things without self driving cars. And then again do we actually need fully self driving cars or is it not just enough to augment the cars that we have because they can have self braking features that take care of pedestrians and we can build a lot of these features into regular cars. Or is it really that we want these cars where we can just hang out and read on our iPads or iPhones and not look into traffic because that's a utopian vision that we might not be anywhere near realising.
Sandra: As we've discussed before on this podcast, getting self driving cars in the middle of Sydney's CBD is very very far away.
Kai: So we're looking forward to what will happen in Manhattan in this experiment.
Sandra: So now for the last scary story. (Robot of the Week audio). The Octopod Clock.
Kai: It's not from the ABC Octonauts children's TV series.
Sandra: It looks like a very high tech octopus. It's got bio mechanical articulated limbs. This bobblehead with a timepiece in it and it looks like something that's a cross between a science fiction movie and a creature that will - what did Gizmodo say?
Kai: It provides a countdown for when it will leap on your head, rip your face off, and impersonate you at parties. And while there is no evidence in the User Manual the thing sure looks like it can do this.
Sandra: This is a limited edition clock and it's a traditional MB&F design meaning that it's about a 100, 150 pieces alone and it's something that's supposed to be fun.
Kai: Also it sets you back about $45,000 Australian dollars.
Sandra: Before you spend that much amount of money, as watch review website Hodinkee mentions, remember that it's guaranteed to cause the heebie jeebies in three point five to six point one percent of the general population who is afraid of octopie.
Kai: And if you are concerned about your mental health, your health in general or what might happen when this thing rips your face off, we have an announcement to make...
Sandra: For a much less scary event, you can join us for the Future of Health - reimagining healthcare research breakfast series that Sydney Business Insights is running together with the American Chamber of Commerce on the 7th of November. We will include all the details in our show notes.
Kai: And that's all we have time for.
Sandra: Hope to see you there. That's all we have time for this week.
Kai: Thank you for listening.
Sandra: Thanks for listening.
Sandra: This was The Future, This Week made awesome by the Sydney Business Insights team and members of the Digital Disruption Research Group. And every week right here with us our sound editor Megan Wedge who makes us sound good and keeps us honest. Our theme music was composed and played live with a set of garden hoses by Linsey Pollak. You can subscribe to this podcast on iTunes, Soundcloud, Stitcher or wherever you get your podcasts. You can follow us online, on Flipboard, Twitter or sbi.sydney.edu.au. If you have any news that you want us to discuss please send them to sbi@sydney.edu.au.
Close transcript