Sandra Peter and Kai Riemer
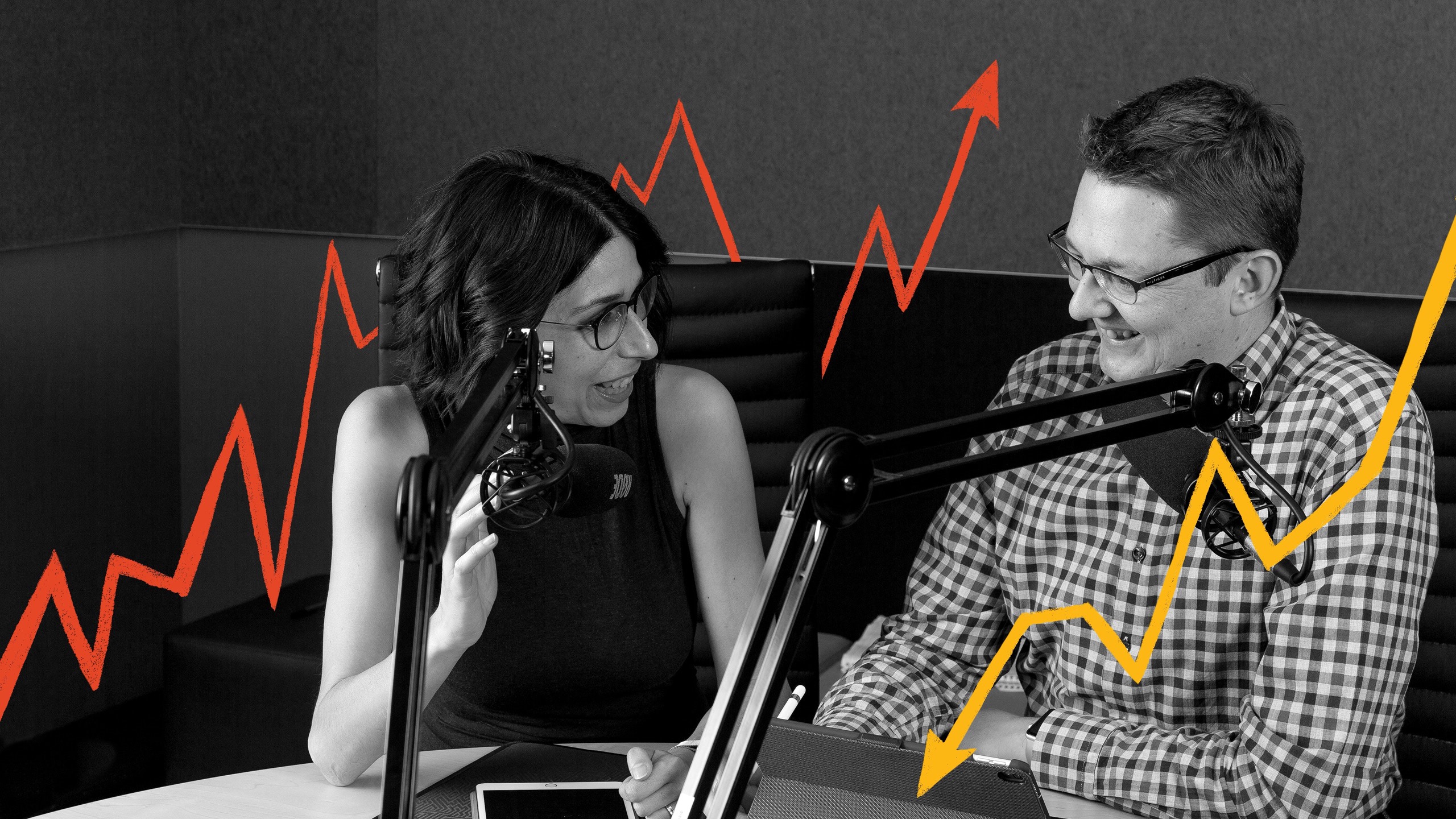
The Future, This Week 1 Mar 19: data is not oil, dangerous AI, and robo-monk
This week: why data is not like oil, dangerous AI, and a robot that gives sermons. Sandra Peter (Sydney Business Insights) and Kai Riemer (Digital Disruption Research Group) meet once a week to put their own spin on news that is impacting the future of business in The Future, This Week.
00:45 – Why data is not the new oil
19:09 – An algorithm for fake news automation
32:34 – Robot of the week: Android Kannon, the AI monk
The stories this week
New AI fake text generator may be too dangerous to release
Other stories we bring up
The Economist argues the world’s most valuable resource is no longer oil, but data
Facebook obtains deeply personal data from Apps
Our previous discussion on TFTW of DNA data sharing
Language models are unsupervised multitask learners research paper
How OpenAI’s text generator works
The Wired story covering the AI fake text generator too dangerous to make public
Our previous discussions of fake stuff on TFTW, including fake reviews and deepfakes
Microsoft’s politically correct chatbot
Robot of the week
The Buddhist robot that gives sermons at an ancient Japanese temple
You can subscribe to this podcast on iTunes, Spotify, Soundcloud, Stitcher, Libsyn, YouTube or wherever you get your podcasts. You can follow us online on Flipboard, Twitter, or sbi.sydney.edu.au.
Our theme music was composed and played by Linsey Pollak.
Send us your news ideas to sbi@sydney.edu.au.
Dr Sandra Peter is the Director of Sydney Executive Plus at the University of Sydney Business School. Her research and practice focuses on engaging with the future in productive ways, and the impact of emerging technologies on business and society.
Kai Riemer is Professor of Information Technology and Organisation, and Director of Sydney Executive Plus at the University of Sydney Business School. Kai's research interest is in Disruptive Technologies, Enterprise Social Media, Virtual Work, Collaborative Technologies and the Philosophy of Technology.
Share
We believe in open and honest access to knowledge. We use a Creative Commons Attribution NoDerivatives licence for our articles and podcasts, so you can republish them for free, online or in print.
Transcript
Disclaimer: We'd like to advise the following program may contain real news, occasional philosophy and ideas that may offend some listeners.
Intro: This is The Future, This Week. On Sydney Business Insights. I'm Sandra Peter and I'm Kai Riemer. Every week we get together and look at the news of the week. We discuss technology, the future of business, the weird and the wonderful, and things that change the world. Okay let's start. Let's start.
Sandra: Today on The Future, This Week: why data is no oil, fake news automation, and a Buddhist robot. I'm Sandra Peter, I'm the Director of Sydney Business Insights.
Kai: I'm Kai Riemer, professor at the Business School and leader of the Digital Disruption Research Group. So Sandra, what happened in the future this week?
Sandra: Lots Kai, lots. It turns out data is not the new oil and AI fake text generators are too dangerous to release out in the wild.
Kai: And finally we'll present you with this week's robot, a Buddhist robot. A robot that can lead a congregation in sermon.
Sandra: Our first story this week however, comes from Wired Magazine and it's titled 'No data is NOT the new oil'.
Kai: Well hang on there. We all know that data is the new oil and here's Kai-Fu Lee the CEO of Sinovation Ventures explaining it to us.
Audio - Kai-Fu Lee: Data is the new oil and China is the new Saudi Arabia. So China has all the data, not only more people but more depth, because so many services are digitised.
Sandra: Kai-Fu Lee is of course also the former president of Google China. And he is not the only one saying this, we've had the New York Times talking about how data is the new oil in the context of the Facebook privacy scandals, we've had The Economist dedicate entire articles discussing that the world's most valuable resource is no longer oil, but data. And of course, we have had Wired Magazine itself talking previously about the value of data and the value of collecting vast amounts of data.
Kai: So likening data to oil obviously implies that it's now one of the most valuable resources, valuable ingredients in doing business. But as the author of the article Antonio García Martínez points out, that comparison actually breaks down fairly quickly when we look at what oil as a resource does and how data behaves and how indeed data is very different from oil in the first place.
Sandra: So 'data is the new oil' is one of those things that's now just generally accepted as being true. So we thought, first thing we should do is let's unpack this. In what ways is data like oil?
Sandra & Kai: Or is it?
Kai: Exactly. The first point that the author makes is that oil is a commodity. Oil is a commodity in the sense that it can be bought on the spot market. There's a few qualities but when you buy oil, you have oil, you can use oil, there's a price for it. You can onsell it and it's still oil. While with data, that is a fairly different beast. So, he makes the argument that if Amazon put all its past sales data on a bunch of hard disks, and put it in a big truck and delivered it to your house, it wouldn't quite be the same for you, that data, as it would be for Amazon because that data of course is highly valuable for Amazon, as it underpins the way it does business. But taking this data out of Amazon, is not the same, it's not like someone could buy this data and use it the same way Amazon does. It would lose a lot of its value that it has in the context of Amazon. Indeed, it doesn't mean that none of that data would be of value to others, like competitors Wal-Mart and so on so forth. But it certainly is far from being a commodity.
Sandra: In this sense it's really an unhelpful analogy to compare something that is quite transportable, quite transferable, to data that is, you know, functionally abstract, and also not equally useful in contexts other than the ones in which it has been gathered.
Kai: And this is not the only way this comparison breaks down. Let's look at the creation. If you want to have oil, you need to do exploration, you need to build facilities, you need to mine oil. It is highly costly to actually produce oil which you can then sell, whereas a lot of the data that we're talking about is just a by-product of activities that either the business engages in when, you know, transactions are being generated, people buy from Amazon, or that people engage in when Facebook collects data about people's activities, what they like, what they post.
Sandra: It's technically digital exhaust, old traces that you leave, and as you interact with your apps on your phone, as you engage with social media, with maps, as you purchase goods and services, all of it generated without you actually making an effort, and with little effort on behalf of the organisations that collect this data to do so.
Kai: Absolutely. And oil is oil is oil, but data is not necessarily data, not all data is the same. So the article for example makes the point that some data, like pictures we post on Facebook, or the posts themselves, might not necessarily be valuable to Facebook in the sense that it tells you all that much about what you're interested in, but it just feeds the feed and it keeps other people interested and it makes the platform more sticky so that it keeps people engaged. Whereas, what you like, you know, the apps you use. Some of that information feeds directly into building your profile. So even on platforms such as Facebook the data is not the same. But what I want to point out is that some data retains its value over time while other data can age fairly quickly. So, if you have location data that you want to utilise to present someone an ad for example, then that data is valuable to you in real time. But as the person moves along the data points become less and less valuable. As behaviours change on the platform, the data pool that is being collected ages over time. And so, if I was to obtain Facebook's data from five years ago it wouldn't be nearly as valuable as the data that Facebook collected today.
Sandra: And yet there is some data that will retain its currency for long periods of time, and I think this is another aspect that we could discuss, and in this way maybe data is a bit like oil. Quite often, all the conversations around data revolve around the fact that this is the data that you collect through social media or location data. But what about DNA data for instance. We spoke previously on The Future, This Week about 23andMe or Ancestry.com, or even open repositories that have mapped the genome of millions of people, that now hold that data and that is not only valuable to infer things about the people who have generated that data, but also data about their relatives or their children, that can be then used to make either policing decisions, or to inform insurance decisions and so on. And in this respect that data doesn't age at all, and it has actually value beyond the context in which it has been collected.
Kai: Also, I can break up oil in discrete quantities. So, you know, I have a million litres of oil, I can sell half of it, and retain half of it. With data, it's not quite the same. So, for example an individual user’s data might not be of much value, but when that data is combined with other users, and groups, and contexts, and communities, the value exponentially becomes more useful and more valuable to the company. If you were to cut this in half, and all of a sudden you only had half the data of that community, the value of that data would be diminished by more than just halving it. So, for all intents and purposes data behaves very differently to a commodity, it's contextual, it can age, it becomes more valuable as you combine it and accumulate it.
Sandra: And there is a marked asymmetry between the people who generate it and could even own it, and the people who can actually make use of it when it's aggregated.
Kai: Yes indeed. So, for example, the transaction data that Amazon collects about me. It's nice for me to know what I have bought from Amazon, but that's just historical data and, you know, it's good to know that I can look this up. But Amazon can do much more with this data. It really becomes valuable to them.
Sandra: Exactly. So the data that you have is much more valuable to a company like Google, or to a company like Facebook that can aggregate that with other data that they collect from their other users, and then can monetise that by allowing advertisers to reach you for instance. Which is way more than what you could do with your own data.
Kai: So, that then brings us to the question - who should own this data? And should I be paid for giving up that data, should Google or Facebook have to pay me for this data, because of course it's very valuable to them. Why shouldn't I benefit or partake in the proceeds? And, you know, shouldn't I be getting what's often called a 'data dividend'?
Sandra: There's a number of ways we could look at answering this. And the answer that is most often discussed, and that we've also had a number of interviews here on Sydney Business Insights, has been yes of course people should be paid for that data, and it's intuitively as easy and as right as 'data is the new oil'. Let's first look at the argument that the article makes, which is how much money would your data be worth, how much would you get paid? And let's remember that if you look at oil dividends, Alaska pays nearly sixteen hundred dollars per person. Saudi Arabia certainly pays a lot more than that to its citizens, as an oil dividend.
Kai: But, Wired did a count of the Facebook global citizenship, and your data is worth about 25 dollars in a year. It's a bit more if you're in the US or Canada, it's about 130 dollars. "Don't spend it all in one place", they say. The point being that individually that data isn't worth all that much, and that goes back to the fact that not all of this data that you create is immediately useful, and also that individually that data is not necessarily all that valuable, or it's very hard to put a value on that data initially, because it becomes valuable as it is being used later on.
Sandra: And let's not forget that most of these organisations actually aggregate data from a number of sources, so it's not just the individual data that you generate on the platform. We've looked in previous episodes at a number of companies that would sell this kind of data and that then a company like Facebook or like Amazon would aggregate and then produce insights out of.
Kai: And we've just seen in a recent article last week that Facebook has been in the news again, as they've been swooping data from apps that use Facebook analytics service, data about women's fertility cycles, who used an app that was not in any way related to Facebook, for their family planning. And a number of other apps from which Facebook obtained user data, data about user activity, which they can then combine with the data that they are collecting anyway.
Sandra: So this to me raises two types of questions. One is, actually is this the right question to ask in the first place? After all, if you are using Facebook you are still using a free service. So the moment they would start paying you, you would have to start paying them as well. Because now that is an exchange, whether that's fair or unfair we could also debate. But you are using a free service, and that is the same with a number of other apps that collect data. The second is, you mentioned things like family planning. A lot of that information is the type of information that you might not be willing to share with any organisation, if you are aware that that is being collected.
Kai: And that is frankly what shits me the most about this argument, because to say 'oh yeah, you know, if you give up that data you should be paid for it'. I think most of the data, or a lot of the data that Facebook and these platforms use to run their business model, you know regarding advertising, they don't want people to know that this data exists in the first place. The extent to which user activity is being tracked. So it does not become a question of whether I'd be willing to sell this data, or should I have ownership of this data. I wouldn't want this data to exist in the first place.
Sandra: So, examples of this, we have seen period trackers that share very intimate information about women. We've seen meditation apps that might have, you know, specific practices for if you're stressed, if you click on that, or if you're depressed that would be sharing very sensitive information about your well-being or your mental health that could be disclosed to say insurance companies.
Kai: Let's not forget that Google's algorithms pass through your Gmail text, and try to sell you advertisements that correlate with the topics discussed in e-mails. People simply wouldn't give consent to actually sell access to this information if they were asked to do so.
Sandra: Which then raises the interesting point of if you were to monetise this data, who would actually disclose it. Because we can make an argument that we might be able to afford not to disclose it, and pay, let's say, a monthly fee to access Facebook or to access certain Google services. But indeed this could be another case of trying to provide some sort of remuneration in exchange for data on people, would create further inequalities in which some people would have to disclose that information in order to gain access to these services Whereas other parts of the population, much better off parts of the population, would be able to maintain their privacy and to access services without the need to disclose the information.
Kai: And we've made this argument previously. Privacy in that instance would then go from a fundamental human right, to a commodity that is being traded, and something that you either can afford, or cannot afford to have.
Sandra: So to sum up, data is not like oil. But here on The Future, This Week we actually quite like metaphors, and we think they're quite useful in structuring how you think about things. So let's hear from Yuval Noah Harari who spoke at Ted last year, on the back of his latest book '21 lessons for the 21st Century". And he is of course a historian, an academic who's also written a number of popular books such as 'Sapiens: A Brief History of Humankind': and 'Homo Deus: A Brief History of Tomorrow'.
Yuval Noah Harari: In ancient times, land was the most important asset in the world. Politics therefore, was the struggle to control land. And dictatorship meant that all the land was owned by a single ruler or by a small oligarchy. And in the modern age, machines became more important than land. Politics became the struggle to control the machines, and dictatorship meant that too many of the machines became concentrated in the hands of the government, or of a small elite. Now data is replacing both land and machines as the most important asset.
Kai: So this is an interesting comparison, to liken data to land, the most valuable resource that any one party could possibly control. And it's a very pertinent comparison and it goes back to the Wired article which also makes the point that it is really the tech giants who collect, amass, and own data, who are not necessarily willing to actually give up that data or trade that data, but rather want to control this data because it is in monopolising the data that the power ultimately lies that these companies can wield.
Sandra: So data not as a commodity, not as oil, but as the most important asset that any one party could control at any point.
Kai: So, the point then is that you don't want it to be like a commodity, right? You don't want it to be like this thing that is the same for everyone. These companies want data to be specific, contextual, relevant to their business, and the focus then, if data is not like oil, a commodity, but like land, like an asset, the focus then is not on trading it. It's not on creating and selling data, but on controlling data, on owning data, on making it relevant, on exploiting it, and amassing a lot of it to then be in a position that we can control, like the advertising market for example, or e-commerce, like in the example of Amazon.
Sandra: And these are all points that Harari goes to some lengths to explain. Because if you're talking about ownership and exploitation, once you concentrate that in the hands of a very small number of people, or indeed single individuals, think monarchies if we're talking about land, people will at some point rise up and try to rectify that imbalance. And we've seen that with monarchies around the world toppling. We've also seen this in the case of capital, in the case of ownership of means of production, we've seen communist revolutions, or where we've seen the emergence of democracy, of people wanting to access, to take part in both the ownership and the exploitation of some of these assets. What we're seeing however in the case of data, and this is quite interesting and we are yet to see how this will play out. And Harari makes this point as well, is that because this process is a lot less transparent and because it affects us in different ways, people will find it much more difficult to challenge established practices and to challenge giants like Google and Facebook.
Kai: But it is nonetheless a fairly grim prediction to say that if this concentration of control, ownership, and exploitation continues then there will be some pushback. Whether this will be by the users, who might abandon platforms or who might not want to give consent, or indeed by regulators. And we've seen some of the beginnings of this in Europe with new legislation around data collection practices. And we don't know where this process is going, but the point that we're making is that it is the kind of metaphors that we're using to talk about data that will allow us to actually make those points. And it is here that the oil metaphor isn't really helpful because it suggests that it is just something that is fluid, that can be traded, that is as uncomplicated as oil as a commodity. Whereas the land metaphor allows us to see in a much better way the role that data might play in many of the problems that we've been discussing with these mega-platforms.
Sandra: And this is a good point to move to our next story because that actually talks about how vast amounts of data can be exploited.
Kai: This has really been all over the news. We've picked one from The Guardian titled 'New AI fake text generator may be too dangerous to release, say creators'.
Sandra: So the story reports on OpenAI. And OpenAI is a non-profit artificial intelligence research company, and behind it are the big names, there are the Elon Musks, and there's Microsoft behind it, there's Amazon Web Services, Y Combinator research, Infosys, that's the Indian multinational I.T. company, and OpenAI has developed the system called GPT 2, successor of course of GBT, that can generate fake text. So it's artificial intelligence that is capable of generating believable coherent pieces of text that are virtually indistinguishable from those written by a human being. So, think deep fakes for text. Before we give you a sample of this, we will just say that the company has taken the unusual step of not releasing their research publicly. Let's remember, OpenAI the non-profit research company's entire goal is to democratise some of this research so they publish everything that they research, they make it available to the general public. But in this case, they have chosen not to do so.
Kai: So before we talk about why they chose not to release this research, because they say it's too dangerous. Let's take a look at what this algorithm actually does.
Sandra: So what do you start out with is giving the interface a sentence to get it going. In this case, the Guardian provided the following fragment "Brexit has already cost the UK economy at least 80 billion pounds since the EU referendum".
Kai: And this is what the algorithm came up with as a story on the back of this first sentence.
Sandra: And the system continued "and many industry experts believe the economic damage from Brexit will be greater still. In some cases the costs will be far harsher. A recent report published by the Royal Society of Arts found that the UK could lose up to 30 percent of its top 10 universities in the future, a move that threatens to push many of our most talented young brains out of the country and onto campuses in the developing world. This is a particularly costly blow. Research by Oxford University warns that the UK would have to spend nearly one trillion dollars on post Brexit infrastructure to replace the lost international talent from overseas. And that's only the beginning.
Kai: So the point here is that the text created by this AI is coherent, it's believable but it is entirely fake and untrue. It is compiled of its body of eight million web pages that the algorithm was trained on. So you give the interface a couple of words or a sentence, and the engine comes up with a text that reads like it was written by a human, that combines facts some data, some prose, some commentary. And for all intents and purposes sounds like a real news article. Now the authors point out that this doesn't work in every case, it works in about half of the cases, but the quality of text is so good that they opted not to release the algorithm.
Sandra: And before we move any further and try to unpack this a little bit, we want to make it quite clear that this is not just about news. So you could use this for fiction writing, for instance another excerpt that was given to the algorithm was the opening line of George Orwell's 1984. "It was a bright cold day in April and the clocks were striking 13". The system will recognise that this is somewhat vaguely futuristic, and it sounds a bit like a novel, and so it continued with "I was in my car on my way to a new job in Seattle. I put the gas in, put the key in and then I let it run. I just imagined what the day would be like 100 years from now. In 2045 I was a teacher in some school in a poor part of rural China. I started with Chinese history and history of science".
Kai: So the algorithm even recognises the kind of genres that these first few words imply. Now, we need to point out that obviously this AI doesn't think, it doesn't comprehend anything. So what it does is, it uses a large body of text that it was trained on, to then produce these texts. And the way this works is the same as we've discussed in the podcast previously. It's a class of algorithms called generative adversarial networks where one AI will randomly attempt to create a text, and another one that was trained on actual human-created texts will then judge that text and say "Ah yeah, no, that doesn't pass muster". So the other algorithm will then go back and create something else, and back and forth a million or so times. Over time, the texts created by the one algorithm will slowly slowly start to pass the judgment tests, until they are no longer distinguishable from human created text in terms of coherence, style. But of course those texts are random in the sense that what is said in the texts, is indeed fake, it's not true, and it is just something that sounds like, and in terms of the makeup of the language, is like the texts in those different genres. Be it news, be it poetry, be it fiction writing.
Sandra: So let's unpack why is this so dangerous. Because really every single news outlet this week has pointed out how this is spelling out the end of the world.
Kai: And we don't want to spend too much time on the implication that they've created this AI, these robots, they're coming for us. They're so good now at understanding language and comprehending, because that's of course not what is happening here. No, we have not created intelligent beings.
Sandra: That's of course bullshit.
Kai: That is bullshit. So why is this so dangerous. First of all of course, because the texts that are being created are highly believable, they're coherent, and they can be used as fake news stories on social media, and so and so forth, if this technology was indeed democratised, as was the original intention of OpenAI.
Sandra: And we've actually foreshadowed this conversation previously on The Future This, Week. We had a very interesting story that we picked up back in September 2017. So about a year and a half ago, we had a look at a story that was reporting on how AI had learned to write totally believable product reviews, and what the implications of writing fake product reviews was. We went through research that was being done at the University of Chicago that was putting forward restaurant reviews that were not only believable, but also perceived as useful by readers of those reviews.
Kai: And of course we've discussed the problems of fake news online in the context of fake videos, of creating believable real-time animated faces, puppeteering other people's faces. And that really points to the heart of the matter here. And it goes without saying, and I'm saying it anyway, that we will of course put all of this in the shownotes.
Sandra: So while most of the conversation online has been about weaponizing this to write fake news, or weaponizing this to promote conspiracy theories, or affect election outcomes and so on. One of the things that we have highlighted repeatedly in our previous conversations around such technology, have been around the ability to use this technology to flood the channels with content, where the emphasis is not necessarily on the content itself, but on the number of such posts. So in the case of restaurant reviews, this was the ability to generate enough reviews to break the business models that relied on the veracity of the reviews. In the context of elections, we were talking about just the ability to generate so many voices as to raise issues to the top of the conversation, in a certain electorate let's say. If you could believably impersonate ordinary people telling their ordinary stories, then you could shift the focus of the conversation.
Kai: So the main problem is not that this technology creates believable fake texts, because humans can do this. The real worry is that this can now be automated and be done at scale, that this technology could be plugged into and underpin fake profiles on Twitter on Facebook, and could really create massive problems for news outlets, for social media sites. It could invade the political process, at a speed and scale that we haven't actually seen before.
Sandra: So, not only can you now create fake reviews or fake stories, fake novels, fake news, but you could even put a face to them now.
Kai: So we can now create fake, believable faces (and we'll put another article in the shownotes from this week), which points to thispersondoesnotexist.com, which showcases this technology. Similar algorithms that do with faces what we see here with text, and then have those faces become fake people propagating fake opinions about political candidates, using this technology. So this is the real worry here, the worry that we will see a gigantic flurry of fake stuff online which will make it very hard to discern what is real what is fake.
Sandra: And so the race is on, not only to generate the equivalent of spam filters for this age, but also to think about how to protect the business models that rely on this sort of content being hard to generate.
Kai: But let's think about where this technology could potentially be used for good, or be useful in the context of various business models.
Sandra: Because of course every time such a thing comes on the scaremongering goes through the roof. But there are of course instances where such technology could be used for positive purposes. The Wired article reporting on the same story (we'll include this in the shownotes) reports on a startup called Narrative Science, where a professor from Northwestern University that works with such technologies is using it to generate things like business documents or financial reporting, where you do need to translate large amounts of data that you might have in an excel sheet, into something that is more easily accessible or more easily reportable to the public.
Kai: But while in the context of spamming channels with fake news, the quality problems that a system like this might have (remember it's only about half of the stories are truly believable) in contexts where we want to use this for generating useful information, for example creating conversational agents that can hold a conversation, we of course run into problems that we have seen previously, such as that text might be severely biased, or might have racist undertones, might be offensive, and it might turn out that these problems are really hard to fix.
Sandra: Whilst we spoke previously on the podcast, and it was a very long time ago, about Tay, the Microsoft chatbot that was unleashed on Twitter and became racist, had to be taken off shortly after being allowed to interact with the public at large. Microsoft has since tried to create a politically correct version. So, think about a younger sister to Tay called Zo. This became the teenage best friend, with #friendgoals, a downright Shakespearean version of the earlier Tay, which became a highly stereotyped version that would send you senseless gags from the internet. Maybe resent you know solving your math problem, but being there for you to give you advice, but having a very blunt filter when it came to any word that might signal that this conversation might have political overtones, or this conversation might have racial overtones. For instance, when told that someone had had good falafel in the Middle East, she would respond "Let me make myself clear, I'm not going to chat politics with you."
Kai: So, this example lets us once again point out what is happening here. The texts being generated are just re-combinations of words based on how close those words are located in the texts that the algorithm was trained on, and while the results have become really really good, this is the result, not of a quantum leap in the intelligence behind it, or the way these algorithms work, but because the researchers were able to train this algorithm on an unprecedented amount of text, which has led to these improvements in quality. But in order to weed out any problems, it would need human intervention. And that would always come at the expense of the kind of engagement or conversational veracity of these texts being created. So, a problem that is very hard to fix because we are only talking with text re-combination here, not with, you know, truly intelligent beings. Which brings us to...
Robot voice: Robot of the week.
Kai: This one was reported in Geek.com. "Meet the Buddhist Robot That Gives Sermons at an Ancient Japanese temple". "A 400-year-old Japanese temple just tapped an AI monk to attract young worshippers". This guy is called Android Kannon, and was developed by well-known Osaka University intelligent robotics professor Hiroshi Ishiguro. And here is what he, she, or it sounds like:
Audio: AUDIO OF ROBOT SERMON
Kai: So, Android Kannon stands at 77 inches, makes 'eye contact', is pre-programmed with sermons from the Heart Sutras in Japanese, but can also translate verses into Chinese and English.
Sandra: A truly "religious landmark", as the article points out. And this is all we have time for today.
Kai: See you soon.
Sandra: On The Future....
Kai: Next week.
Sandra: This Week.
Kai: Yes, but next week.
Sandra: On The Future, This week. Next week.
Outro: This was The Future, This Week made possible by the Sydney Business Insights Team and members of the Digital Disruption Research Group. And every week right here with us, our sound editor Megan Wedge, who makes us sound good, and keeps us honest. Our theme music was composed and played live on a set of garden hoses by Lindsey Pollak. You can subscribe to this podcast on iTunes, Stitcher, Spotify, YouTube, SoundCloud, or wherever you get your podcasts. You can follow us online on Flipboard, Twitter, or sbi.sydney.edu.au. If you have any news that you want us to discuss, please send them to sbi@sydney.edu.au.
Close transcript