Sandra Peter and Kai Riemer
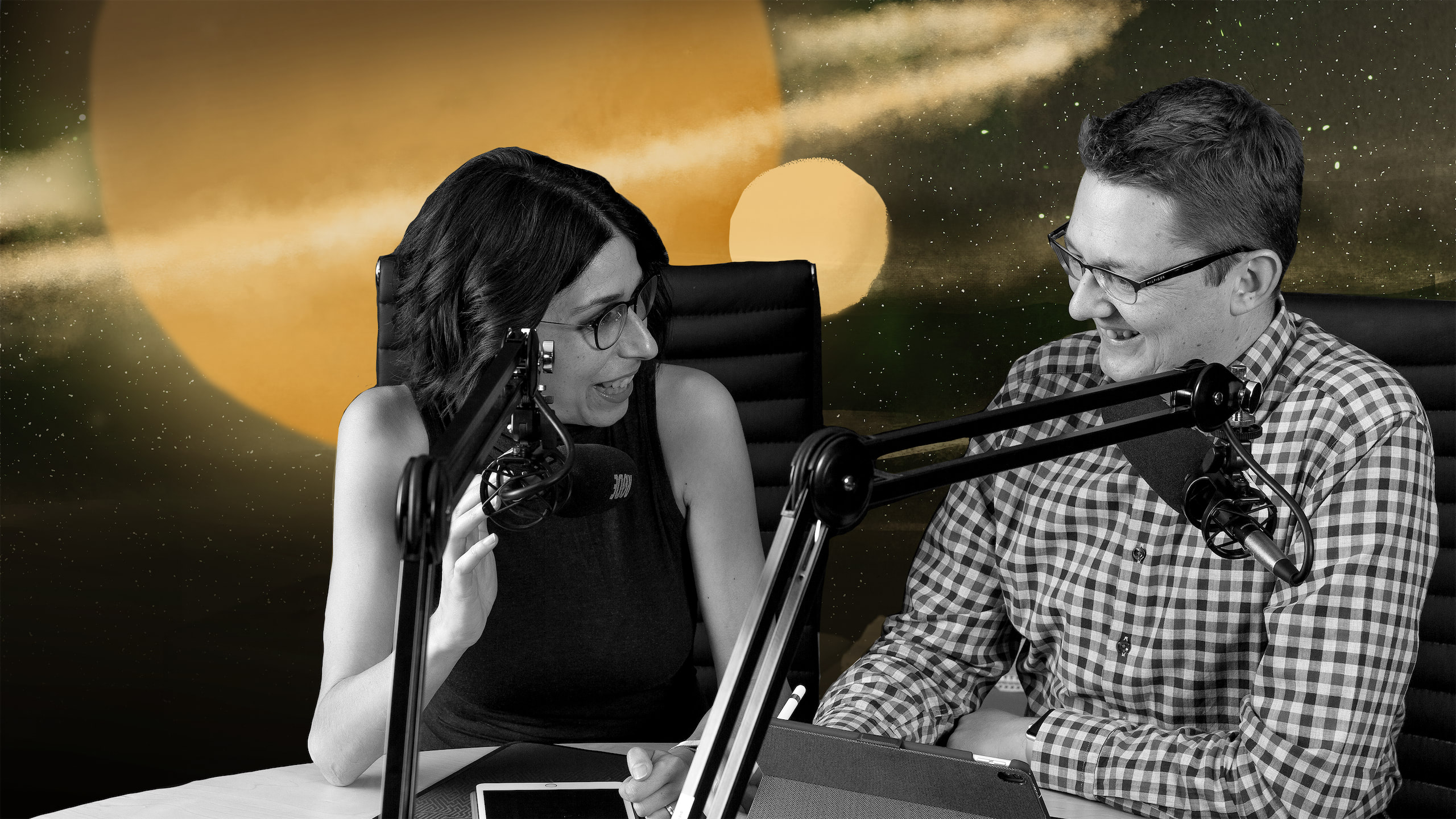
The Future, This Week 28 July 2017
This week: what happened while we were gone, real problems with AI, spying vacuums, and a suicidal robot. Sandra Peter (Sydney Business Insights) and Kai Riemer (Digital Disruption Research Group) meet once a week to put their own spin on news that is impacting the future of business in The Future, This Week.
The stories this week
Elon is worried about killer robots
Roomba, the home mapping vacuum cleaner
Other stories we bring up
Google’s collaboration with Carnegie Mellon University paper
Cathy O’Neil’s book Weapons of Math Destruction
Do algorithms make better decisions?
Roomba data will be sold to the highest bidder
How to Use iRobot Roomba 980 Robot Vacuum
Our robot of the week
You can subscribe to this podcast on iTunes, Spotify, Soundcloud, Stitcher, Libsyn or wherever you get your podcasts. You can follow us online on Flipboard, Twitter, or sbi.sydney.edu.au.
Send us your news ideas to sbi@sydney.edu.au
For more episodes of The Future, This Week see our playlists
Dr Sandra Peter is the Director of Sydney Executive Plus at the University of Sydney Business School. Her research and practice focuses on engaging with the future in productive ways, and the impact of emerging technologies on business and society.
Kai Riemer is Professor of Information Technology and Organisation, and Director of Sydney Executive Plus at the University of Sydney Business School. Kai's research interest is in Disruptive Technologies, Enterprise Social Media, Virtual Work, Collaborative Technologies and the Philosophy of Technology.
Share
We believe in open and honest access to knowledge. We use a Creative Commons Attribution NoDerivatives licence for our articles and podcasts, so you can republish them for free, online or in print.
Transcript
Introduction: This is The Future, This week on Sydney Business Insights. I'm Sandra Peter. And I'm Kai Riemer. Every week we get together and look at the news of the week. We discuss technology, the future of business, the weird and the wonderful things that change the world. Ok let's roll.
Kai: Today in The Future, This Week: what happened while we were gone, real problems with AI, spying vacuums and a suicidal robot.
Sandra: I'm Sandra and I am the Director of Sydney Business Insights.
Kai: I'm Kai Riemer, professor at the Business School and leader of the Digital Disruption Research Group.
Sandra: So Kai, what happened while we were gone?
Kai: Well first of all we're back. So this is the second season of The Future, This Week and we want to start by catching up on some of the themes of the stories that we missed while we were away on break. Sandra was travelling, I was travelling.
Sandra: And whilst we had the short segments that were pre-recorded on a few topics that we never had time to get to. Quite a few things happened over the last few weeks and we're gonna get to some of them today.
Kai: Yes so obviously there's too much for us to cover today but let's highlight a few themes. Now first of all there was a whole lot of things on AI and we're going to discuss some of it today. We're back but who else is back?
Sandra: Elon Musk is back.
Kai: Elon Musk is back and the killer robots are back in the warnings and we're going to discuss this. But there was other things on AI, on robots automation, and there's a noticeable shift in the conversation now. The hyperbole is kind of retreating a little bit and as we predicted some more reasoned voices are coming in, some more balanced views as to the role that automation, AI, and robots will play in augmenting our jobs rather than necessarily getting rid of all our jobs.
Sandra: And today we will talk about those more reasoned voices. Unfortunately there seems to be not a lot of interest neither from government nor from the companies involved in their development in listening to some of these more reasoned voices and what they're saying.
Kai: Yeah there's other themes. Wearables have made a comeback as a topic. There's been a whole discussion around wearables are dead. No they're not. Google Glass is making a comeback. Intel is out of the picture. Apple is updating the operating system for the Apple Watch. So there's mixed messages there. But it seems that while some people are dismissing it, others have found new ways of actually employing wearables in interesting ways. So that's a topic for the next couple of weeks.
Sandra: Robots are also back. We've spoken a lot about robots in the first season of The Future. This Week, all of which you can listen to on SoundCloud, iTunes, Stitcher or wherever you get your podcasts from. But robots have made a comeback again. They're stealing our jobs, they're not stealing our jobs, they're stealing our jobs a little bit slower than we expected, they seem to be stealing different jobs than we expected. So will rehash that conversation and try to explore a little bit more in depth what the real questions are.
Kai: And some really interesting articles that highlight that the whole discussion around the future of work and automation is not a new one right? So going back to the 1800's, the role of technology, the fear of technology. So we're going to go back in history and show that the narrative that we are experiencing at the moment is by no way a new one. It's been as old as modern technology.
Sandra: So 500 years of tech stealing of our jobs.
Kai: Absolutely. And then finally there's been a whole bunch of articles around cars. Now there was Volvo making the announcement that by 2019 all of their cars will have some electric component hybrids or fully electric. And that in the future they're going to phase out petrol based engines entirely. So a discussion around the fact that electric cars are maybe coming quicker than we thought. But at the same time there's still problems with self driving cars and a little bit of scepticism as to whether they are actually coming as fast or at all in the way in which some of these companies are envisioning it.
Sandra: And we are definitely going to cover some of this less sexy topics things like electric vehicles - everyone's talking about autonomous vehicles. But there's actually quite a bit to discuss here. So we'll come back to all of these topics over the next few weeks.
Kai: New energy. We've talked batteries for quite a bit in the first season. Solar Sustainability, Energy Revolution, solar technology all of these kind of things will come back because we're seeing some real movement in those markets with implications not just for these market themselves but also the business, landscape, society and the whole discussion on climate change more generally.
Sandra: And also a whole bunch of other stories that take us around the globe as well with Chinese companies that are going into Indonesia into a whole host of other countries investing in the very AI that we spoke about at the beginning. Instagram reshaping restaurants from Hong Kong to Singapore, solar power rays that look like pandas and the Internet of sheep, tech waging wars on disease carrying mosquitoes, umbrella sharing companies that are struggling to stay above the water.
Kai: India overtakes the US to become Facebook's top country, free robot lawyers help low income people take legal issues.
Sandra: Robots even hunting lionfish in Bermuda.
Kai: And Estonia, a country run like a start-up.
Sandra: So clearly there's a whole lot we could talk about. We're going to focus on a couple of things today.
Kai: So Sandra what happened in The Future, This Week?
Sandra: Our first stories from Wired, asking Elon Musk to forget killer robots and focus on the real AI problems. Recently Elon Musk with 50 of the most powerful politicians in America at the National Governors Association meeting and he told them again that the biggest threat to humanity is actually robots killing us.
Kai: Yeah. So when you long Musk talks about how AI presents a fundamental threat to human civilization, he is really envisioning straight out of the Robocop and Terminator playbook. The uprising of the machines, robots hunting humans and destroying civilization. So it all sounds pretty gloomy. Let's hear from the man himself.
Audio: I think people should be really concerned about it. I keep sounding the alarm bell. But until people see like robots going down the street killing people they don't know how to react. You know because it seems so ethereal. And I think we should be really concerned about AI, and I think we should, AI is a rare case where I think we need to be proactive in regulation instead of reactive. Because I think by the time we're reactive in AI regulation, it's too late.
Sandra: So we see a small problem with his apocalyptic predictions and it's not just us. It's most of the rest of the world and people actually developing this technology.
Kai: Yeah Mark Zuckerberg for example has come out and said look you know I think this is overblown, I don't share this. I'm more optimistic and he's been criticized by Elon who says Mark Zuckerberg really doesn't understand AI fully, I understand it and it's terrible and these things are a real threat. But you know others are not quite as convinced so the Wired article for example sites Pedro Domingos, a professor at the University of Washington who works on machine learning and who knows what he's talking about and he basically says many of us have tried to educate him about real versus imaginary dangers of AI but apparently none of it has made a dent. So the point that they make is that current technologies and machine learning and pattern matching, they are far far away from achieving the kind of artificial general intelligence that Elon Musk is afraid of.
Sandra: So before we go into the real problems of AI and we've discussed this on the podcast before, so what's the difference between the type of AI technology that we have today, the specialized kind and the generalized kind of intelligence that Elon Musk is afraid of.
Kai: OK. Well on a basic level what Elon Musk is afraid of is that machines develop the kind of intelligence that humans have where they can actually think beyond a task that they've been given, and form their own plans and basically develop some form of consciousness and reasoning and therefore desires. Those desires might actually be harmful to civilization.
Sandra: So instead of recognising kittens in pictures it will actually capture kittens to take their picture.
Kai: The problem with that is that this is a completely different ball game to what we have at the moment, like take identifying cats in pictures, right? While we have algorithms that can quite reliably identify cats in pictures those algorithms have no appreciation of what a cat is. Right. They have no understanding of what a cat is. They can't interact with cats. They don't know cats, nor do they care. No nor do they care. They do not live in this world. They don't have a stake in this world. They are just algorithms that can identify patterns that to us look like cats. They wouldn't know what they're looking at. They just can reliably identify the thing that we call a cat. To assume that this form of pattern matching it, it can be very sophisticated it can work at scale it's fast and it can be used for all kinds of things. But to assume that this is akin to any kind of intelligence that we have that allows us to actually live in this world and do all kinds of purposeful things is ludicrous. And people are pointing this out. So to assume that we are on a path way of you know if we only improve these technologies we will achieve artificial general intelligence, there's really very little reason to believe that this is possible.
Sandra: Or that we understand the mechanism by which we will get there because the other assumption that Elon makes is that we could stop this through regulations because we clearly understand how this will develop out of the technologies that we have now. Whereas for the this to happen we actually need different types of technologies, different types of algorithms, different thinking that would get us that which we don't.
Kai: Absolutely. And I would argue that we would actually have to do the opposite not learn more about technology but actually engage with what human intelligence is like. We don't even have an understanding of how consciousness arises in the brain nor do we fully understand or grasp what it means to live a human life and the human existence and how this all connects up with the neurones in our brain. So in my view in order to make any progress in that respect we would have to heavily study human intelligence in the first place. But that's not the main point of this article. The main point of this article is that this discussion around the dangers of artificial general intelligence is a distraction. What we should be focusing on is the real dangers that come from the pattern matching algorithms as they are unleashed on the world today.
Sandra: So for us this raises two important questions: first that it gives disproportionate power to a few large tech companies in a more sophisticated way than maybe some people realize or understand. And secondly, they unleash a whole host of biased algorithms that a lot of people don't know, don't understand, but more importantly a lot of companies don't care about.
Kai: So that ties in with two other articles that appeared while we were away. The first one again in Wired, called "AI an enormous data could make tech giants harder to topple". So the argument made there is that some few companies which we refer to as the 'Frightful Five' previously, are in a position to actually be the dominant players when it comes to AI because as a study shows that's mentioned in the article, if you have access to enormous data and the example shows that makes a difference whether you train an algorithm with a million pictures or 300 million pictures, you're actually in a position to make your pattern matching algorithm more accurate.
Sandra: So what the article talks about is a new paper that Google has released that reports on a collaboration that they have with Carnegie Mellon University where they use image recognition experiments, so think about the kittens that we just discussed were recognising cats in pictures. But what they did is that instead of using the million images that you would normally use that are labelled cat and you recognize the cat, they actually use. 300 million labelled images. So going from what we describe as really big datasets to enormous datasets and this would be something that only data rich companies like Google or like Facebook or Microsoft would actually have access to. And what this actually produced and this is reported in a paper that's now widely available, what this was designed to do was to test whether the existing algorithm could do a lot more with a lot more data. And the answer to the question was yes actually it can, it produced an improvement of three percentage points.
Kai: So you could say 3 percent that's not much right?
Sandra: Yes but the question is 3 percent of what?
Kai: That's right. So if you have an improvement in your margin or your turn of 3 percent that can be billions of dollars especially for these large companies.
Sandra: So I think advertising, think retailing or just think the volume that companies like Google or Facebook...
Kai: Conversion of leads into buying customers, all these kind of things.
Sandra: It also means that it creates huge barriers to entry. So you might have smaller companies with a smarter algorithm with a better way of thinking through the problem. But they will never have access to the type of data they need to actually train their algorithm to get better at what it does.
Kai: And so the article makes the point that it puts into perspective the fact that some of these companies have released their AI algorithms for everyone to use. The point they make is they can do this they can freely open source the algorithms because without access to this enormous data they don't pose a competitive threat to the power that these large companies can generate from their data as they feed them into their machine learning algorithm.
Sandra: So the two options for some of these small companies as it stands now would be one to pull together their resources with other small companies that would have this type of data. And the market incentives sometimes are there, sometimes are not there around freely sharing the data because it spreads the advantage but more often than not these companies end up being acquired by the very giants that they're trying to displace and we clearly have examples of this happening in China which is also very very active AI market.
Kai: And so the article makes the point that while there might be profitable new issues for smaller players entering the AI field. There's a real danger a real risk that the application of AI might further cement the power, an uncontrollable kind of monopolistic power by a few large companies that are very hard to control, very hard to regulate. And that might lead to other problems such as bias.
Sandra: Yes. Which brings us to the other story that we have, a story that comes out of MIT's Tech Review, which talks about the fact the biased algorithms are everywhere and that no one seems to care. Now the problem with these buyers models is that they end up remaking our lives and the companies that are responsible for developing these algorithms, so for instance the Googles and the Facebook and the Microsoft of the world have no real incentive in being very careful about what they do. Sometimes these very biases give them a competitive advantage. Nor is the government interested in addressing these problems at the moment. There is no real benefit in addressing it and quite often we don't know exactly how to address the problems that we have.
Kai: So in the article Cathy O'Neil who's a mathematician and the author of a pretty great book called "Weapons of math destruction". It's a book that highlights the risk of algorithms. She's making the point that she has created a business that will help companies eradicating or dealing with bias in their algorithms she says "I'll be honest with you, I have no clients right now". So there seems to be very little awareness or interest by these companies and actually tackling the problem of bias in these algorithms. So what are some of those problems that stem from algorithmic bias?
Sandra: There are a number of problems indeed. First there's that in order for us to teach these algorithms we use huge quantities of written or spoken language. And this might introduce biases in the way we put the data in. If there are more men and women speaking in the training content that they provide to my algorithm, than my algorithm will be biased. These AI systems that handle language will then result in chat bots that might be biased, they might result in translation systems, in image captioning systems or in recommendations, so you might have biased recommendations around who should get a job or who should get promoted or how we should rank certain people or certain websites.
Kai: So if we use data on past hiring successes to train our algorithms to make recommendations on which candidates to hire then those algorithms will perpetuate any biases that are already in our past decisions right? So it's often said that we're using algorithms because they're unbiased but that's not true right because they need to be trained in whatever bias is in the training data, will then be in the decisions that those algorithms make. And so the question then is who do we actually entrust with selecting the data to train the algorithms and so thereby this will invariably make it into the algorithm.
Sandra: And quite often their biases might make it into the algorithms and also the data that they have available might introduce those biases because the data we have is also biased in certain ways. And let's remember that these impact the lives of people quite directly, so for instance who gets a job interview. Right now the screening for a lot of job interviews is done preliminary by an algorithm who gets to be granted parole. Those things are screened by algorithms. Who gets to get a loan or who gets to have their business funded. There might be an algorithm behind all of these things.
Kai: Or take image recognition for example. There was a case a while back and I think we mentioned it on the podcast in the first season, a woman from M.I.T, a researcher by the name of Joy Buolamwini, she recognized that face recognition systems systematically had problems recognizing her black face. And she started wearing a white mask so all the computers and the robots in her lap could recognize her. She tells a story that she first came across this problem years and years ago. But would assume that someone would fix these problems. But she says that researchers and developers would use standard data sets for training their algorithms which systematically would have problems recognizing black faces. And so she started this initiative to eradicate bias in face recognition and pattern matching and machine learning more generally. So as we can see quite unconsciously or unwantedly, these biases creep into these algorithms and then create real problems for real people in the world.
Sandra: And of course researchers from Boston University for instance and also research from Microsoft showed us that actually it also happens in more insidious ways. So in this case there is buyers that we can actually recognise because we can see when it is biased towards not a single black face in this case. But for instance the researchers from Boston University showed that dataset that had word programmer in it sold the word closer to man and then to the word woman. And similarly the closest word they found to woman was the word homemaker. So in that case that bias hides itself in the way that those algorithms are then applied. So we saw another professor from Stanford University who is mentioned in the article, who says that they tried to run experiments to see just how far this would go and how would that bias manifest itself. And in this case they had a program designed to read web pages and ranked their relevance and found that the system actually ranked information about female programmers as less relevant than information about their male counterparts. So in this case the bias then becomes hidden within the algorithm.
Kai: Absolutely. And we might argue that yes there's a bias there but where does this bias come from? And the point and I've written about this previously is that, when we use current data about the world to train those algorithms it will tie us into what the world is like today. So it will actually incorporate a certain reality which in some ways might be skewed towards certain groups at the exclusion of other groups. So while it might be true that there are more male programmers than female programmers, we do not want this reality to creep into our hiring decisions for example, we do not want to perpetuate the biases that exist in the world. But the problem is that when we use these algorithms they will invariably tie us in to what the world is like today. So they will perpetuate whatever biases exist in the world if we're not careful. And therefore ironically they will actually prevent certain change from happening. So when we hire people we want to be balanced. We want to not bias our decisions on the basis of gender right, but invariably those algorithms will if we train them on what the world is like today. So they are not able to actually incorporate certain values that we hold but rather mirror the things that we want to overcome.
Sandra: Exactly. And unconsciously removing that bias from the way we do this is actually dangerous in itself as well because these algorithms at some point need to model the real world. So do you skew the modelling of the real world or do you skew than how it makes decisions in the future.
Kai: And then we are faced with the problem that we assume that algorithms can make unbiased decisions. But the data is already by so who then gets to decide what is an unbiased version of reality? Who do we entrust the job of creating an unbiased dataset when the whole point was that we didn't trust people because they are biased. And so we reach for the algorithms which are supposedly unbiased but then it is always people training them. So we shouldn't kid ourselves in assuming that algorithms are unbiased. But the question then is why do we generally put so much trust into those algorithms and in the supposedly superior, more rational, more unbiased nature of a technology driven decision making for example.
Sandra: Cathy O'Neil which we've mentioned before who is the mathematician who wrote "Weapons of math destruction" actually shows that we are biased towards believing that mathematical representations are free from the same sort of flaws that we have. So then people invariably trust these algorithms more than they trust people and actually destroying the blind trust in those algorithms or the blind trust that mathematics are bias free is a much more difficult job than it might seem.
Kai: But also the problem then becomes that once we recognise that those black box algorithms that we have placed so much trust in actually lead to unwanted social exclusion, digital divide, inequalities, lack of access and problems with fairness, down the track people might actually start resisting whole sale the application of these algorithms because they are black boxes, because we placed trust into these algorithms which led us astray or turned out to be misplaced. The article makes the point that if we're not careful and address this problem and be transparent about the biased problems in those machine learning algorithms, there might be a backlash and a wholesale rejection of this technology which might be detrimental to all the good things you could potentially do with the technology.
Sandra: Transparency is especially important in the case of financial companies and tech companies that are predominantly using these algorithms today.
Kai: And this is where the initiative that is mentioned in the article AI now comes into play, trying to raise awareness for the problem the first instance and then engaging corporations in recognizing that there might be systematic problems and then dealing with those biases, a problem which is very hard to tackle. As the article says, because you know a lot of instances the algorithms seemingly work they lead to more efficiency, they lead to more profits and so why should anyone be concerned?
Sandra: Sometimes the bias works for the companies that have developed these algorithms, so quite often the biases are there, but they're actually making life easier for the companies that use them. So there is very little incentives on the part of many of the stakeholders in this process to do anything about it even if they recognise that....
Kai: The problems are often hard to recognise because if we're hiring based on algorithms and there might be a bias in there, we cannot really systematically feed all the data that we would need into the algorithm. For example we do not know where the candidates go that we reject and didn't hire. They might have turned out to be phenomenal candidates but we do not have any data of how well they would have done had we hired them. Similarly with say a loan decision for a small business right, if the algorithms says the likelihood of this business going bankrupt is very high and we don't give them a loan that might actually become a self-fulfilling prophecy. And the company might go bankrupt because we didn't give them the loan, right. So there are systematic problems with the application and the improvement of these algorithms that we first of all have to be aware of in order to then tackle them.
Sandra: Speaking of being aware of things our vacuum cleaners are now becoming aware.
Kai: Our next story is from Reuters magazine titled "Roomba vacuum maker iRobot betting big on the 'smart' home".
Sandra: So what's happening here are Roomba's becoming even smarter? Are they taking over the home?
Kai: iRobot's CEO Colin Angle has laid out his vision for what the company will become besides a robot maker that creates vacuum robots. And it's about the data that the little thing collects when it vacuums our homes as in it maps out where the furniture is and it gains a pretty good approximation of the layout of the home, what each room is for while it uses its sensors to figure out how to do its job.
Audio: A full suite of sensors and a visual localisation system help the Roomba 980 map your home as it cleans so it always knows exactly where it is, where it's been and where it needs to go next.
Sandra: So what the Roomba ends up with is this entire set of data that maps out your home. So what could it now do with this data.
Kai: So one thing is to facilitate the creation of the Smarthome. So the article talks about selling this data to companies such as Amazon, Apple, Google you know the frightful five who are selling services for the home in the form of Alexa, Google Home or the newly announced Home Pod by Apple. So you could use this data to improve the positioning of this device and the mapping out could help with you know optimal sound so there's all these visions about how the data collected by the Roomba could tie in with optimising you know acoustics and things like this in the home.
Sandra: So imagine if your sound system actually had a true map of your home when it tried to optimise the sound and of where things have moved around in your home for instance. Same with the smart lighting or with the thermostats that would get an accurate reading of your home and an updated reading of your home. There's an entire ecosystem of devices that we are now putting in our homes that approximate what our home might look like or have a very rough guide. iRobot would be able to basically provide these maps to all of these large companies that could then use their own devices and their own services to provide you with a better Smarthome experience.
Kai: But is it just me or isn't that a little creepy.
Sandra: Just a little bit, just a little bit.
Kai: So there's another article in Gizmodo which makes that point and calls the vacuum cleaner a creepy little spy. So the point being Roomba will have data about the layout of your home which it will potentially sell to other companies and once this data is out there who knows what the data will be used for. So do I want this? Do I want the lay out of my home and it'll know where the child lives because it's where it bumps into things the most. And you know do I want this data to be out there to be used by corporations and potentially be leaked. I don't know who's going to get hold of the data.
Sandra: So on the one hand there are advantages that you could argue based on you giving up your data on the other side as you mentioned it is quite scary for two reasons. One is that not many of us actually read these privacy agreements when we buy our Roomba and think oh my God this machine is mapping my home and selling it to the real estate agent so that they could know whether I've move things around, I've shifted things around and selling it to Amazon so that it can not only optimise the air conditioning flow but also sell me a whole host of things that could improve my life or just make me spend a little bit more money on lighting according to the time of the day and so on and so forth.
Kai: Yeah absolutely. So when confronted with the question of whether customers would be keen on having this data collected Colin Angle the iRobot CEO says oh you know he reckons that most customers would agree to doing this but when he says that he probably means me clicking I agree when I sign up to the service and this message pops in front of me and I'm not reading the 15000 word agreement where somewhere on the 15th page there's a paragraph about the data being shared with third parties. So the pushing of the button I agree is that enough consent for this kind of data? That's the question here.
Sandra: And it doesn't end here right? You could collect a lot more data than this.
Kai: Of course Roomba could use sensors to analyse all the kind of dust and shit it pops up. And in the name of creating services that will provide you with an assessment of the allergenic content of your home in the name of health and fighting allergies we could devise more services that collect more data but then again...
Sandra: Which on the one hand could make my life a lot better. But on the other hand...
Kai: Once you have this data and you do a chemical analysis of the dust in your home that data might be shared with other parties such as you know law enforcement and you know you might be running an Air BnB and have couch surfers in the home and unwanted substances might end up in your dust. A chemical analysis might trigger the authorities in apprehending you.
Sandra: So who knows what this could be used for.
Kai: Absolutely.
Sandra: And clearly this is not restricted to one company. So this is one of the examples where if we think about the type of data and the data advantage that you would get, well actually in a space like the iRoomba you've got Black and Decker, you've got Hoover, you've got all these other companies doing similar devices and they're actually patents are all that are keeping these companies ahead because the amount of data they collect actually doesn't provide them a competitive advantage. I could get the same data from each of them if I'm Amazon or Google - I wouldn't have to restrict myself to the one that is selling now but the cheapest competitor out there.
Kai: So the bigger message here is that again, and we talked about this before, anything in the way of data that is collected, can be shared and can be used and appropriated for other purposes. It used to be our mobile devices that we used out and about in the world but now we have these technologies such as Alexa, Home Pod and vacuum cleaners and sensors and smart lighting come into our own private homes so there's more and more devices that are potentially able to collect data and share this with third parties that are on the very spaces that used to be the last private islands that we could go to and be away from the public.
Sandra: More and more stakeholders involved in this process and also increasingly difficult to disassociate the benefits that we gather from releasing all of this data that we have.
Kai: So harking back to our first story the balancing of what kind of information we collect, the kind of black boxes that we create to provide us with all these new services, but on the other hand the privacy that we might compromise in the process and then the trust that might be lost when this data is being used and abused down the track it makes for an interesting conversation going forward when it comes to the acceptance and the usefulness of all these new services.
Sandra: And something we'll definitely keep an eye on. Let's remember the iRobot came out of military applications for robots that could scalp for basically bombs and now we have them in our homes.
Kai: Which brings us to... [AUDIO: Robot of the Week]
Sandra: Today it's the nightscope security robot at the Washington harbour shopping mall. And this is actually a sad story.
Sandra: The new security robot at the mall was found having drowned itself in a couple of inches of water.
Kai: Now we need to describe this. So first of all Sandra what does this little guy looked like?
Sandra: It looks a bit like a cross between R2-D2 and a suppository.
Kai: Yeah sadly that's true. And we reckon that this little guy somehow was one of the first as foreshadowed by Elon Musk that gained consciousness.
Sandra: And when it realised what it looks like - it's nothing like the Terminator, it has no gun, it doesn't even have arms. It decided that this life is not worth living as a mall cop.
Kai: No it's said oh geez you know look at what I look like what I have become a security guard in this boring mall and it headed straight for the pond poor thing.
Sandra: I think that tweet by Bilal Farooqui summed it up really nicely. It said "our DC office building got a security robot. It drowned itself. We were promised flying cars, instead we got suicidal robots."
Kai: And this is all we have time for today.
Sandra: We'll see you next week. And welcome back to season two.
Kai: Thanks for listening.
Outro: This was The Future, This Week. Made awesome by the Sydney Business Insights team and members of the Digital Disruption Research Group. And every week right here with us our sound editor Megan Wedge who makes us sound good and keeps us honest. You can subscribe to this podcast on iTunes, SoundCloud, Stitcher or wherever you get your podcasts. You can follow us online, on Twitter and on Flipboard. If you have any news you want us to discuss please send them to sbi@sydney.edu.au.
Close transcript