Sandra Peter and Kai Riemer
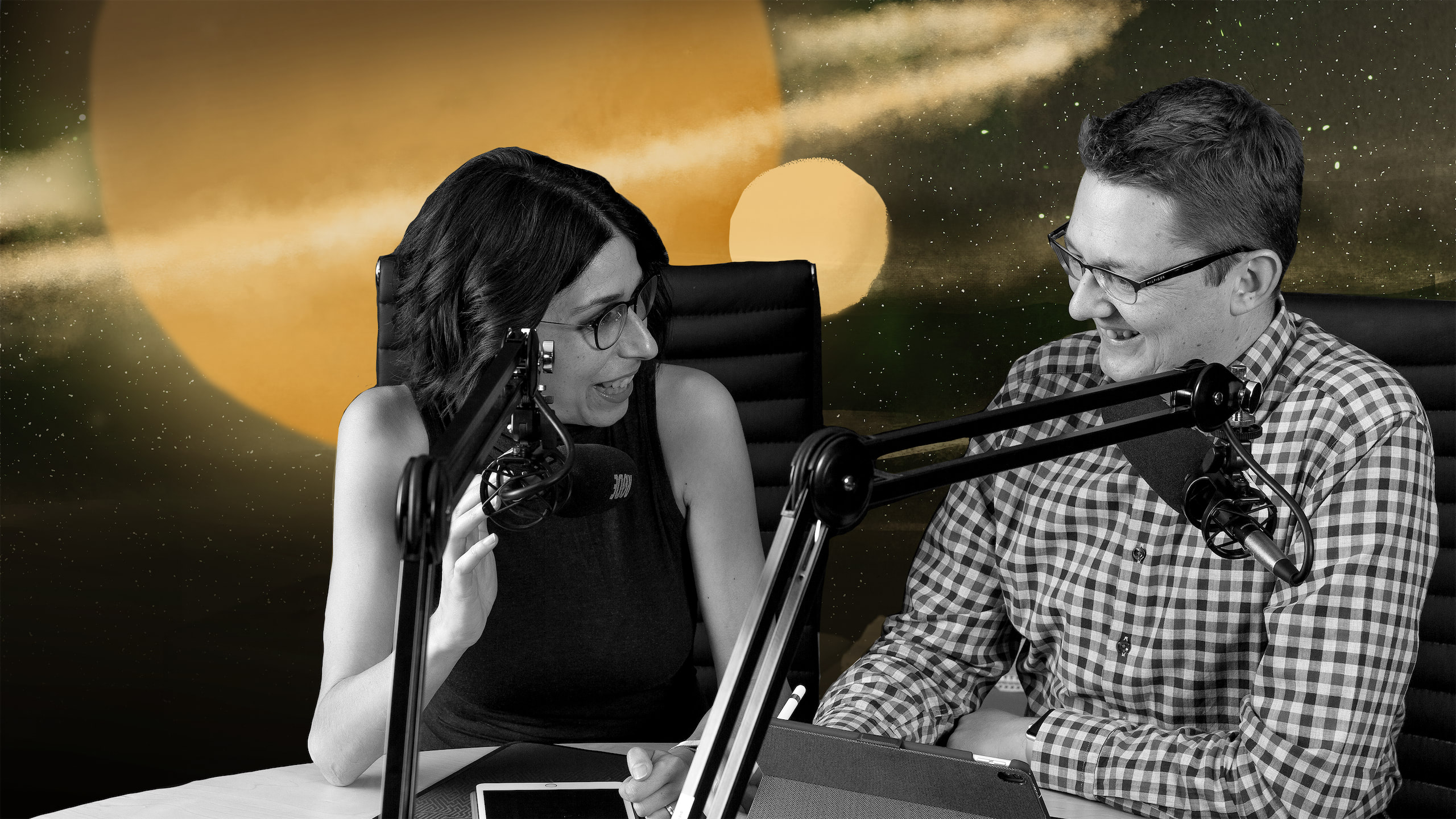
The Future, This Week 17 November 2017
This week: re-cycling data, Watson’s health problems, and shop the look on Spotify. Sandra Peter (Sydney Business Insights) and Kai Riemer (Digital Disruption Research Group) meet once a week to put their own spin on news that is impacting the future of business in The Future, This Week.
The stories this week
How bike share schemes make money
Now you can buy makeup through Spotify
Other stories we bring up
City planners tap into Strava data
Citylab on using Strava data in city planning
Research paper on the use of Strava
IBM Watson versus cancer on Statnews
IBM’s Watson for Oncology website
The Memorial Sloan Kettering Watson Oncology website
Spotify expands to beauty products
How much do artists earn on Spotify?
Artists sharing data on the steaming earnings
The Future, This Week 23 June 2017 (our previous bike-sharing episode)
You can subscribe to this podcast on iTunes, Spotify, Soundcloud, Stitcher, Libsyn or wherever you get your podcasts. You can follow us online on Flipboard, Twitter, or sbi.sydney.edu.au.
Our theme music was composed and played by Linsey Pollak.
Send us your news ideas to sbi@sydney.edu.au.
For more episodes of The Future, This Week see our playlists.
Dr Sandra Peter is the Director of Sydney Executive Plus at the University of Sydney Business School. Her research and practice focuses on engaging with the future in productive ways, and the impact of emerging technologies on business and society.
Kai Riemer is Professor of Information Technology and Organisation, and Director of Sydney Executive Plus at the University of Sydney Business School. Kai's research interest is in Disruptive Technologies, Enterprise Social Media, Virtual Work, Collaborative Technologies and the Philosophy of Technology.
Share
We believe in open and honest access to knowledge. We use a Creative Commons Attribution NoDerivatives licence for our articles and podcasts, so you can republish them for free, online or in print.
Transcript
Introduction: This is The Future, This Week on Sydney Business Insights. I'm Sandra Peter. And I'm Kai Riemer. Every week we get together and look at the news of the week. We discuss technology, the future of business, the weird and the wonderful and things that change the world. OK, let's roll.
Kai: Today on The Future, This Week: re-cycling data, Watson's health problems, and shop the look on Spotify.
Sandra: I'm Sandra Peter, I'm the Director of Sydney Business Insights.
Kai: I'm Kai Riemer, professor at the Business School and leader of the Digital Disruption Research Group.
Sandra: So Kai what happened in the future this week?
Kai: Our first story is from The Sydney Morning Herald. It's titled "How your personal information funds shared bike schemes". So this article reports on the business models of all the bike-share companies that are popping up around Sydney so people who live in Sydney or have been to the city of Sydney recently will have noticed all these red and yellow bikes sitting around everywhere so you can rent those bikes by the hour basically. You sign up to the service on your smartphone app, you can locate your bike via GPS, you can ride the bike and basically leave it anywhere you wish. The company will find the bike and collect it and put it in places where there's lots of demand for it and it's relatively cheap. So the article asks the question how can these companies actually make money. And the also quotes an expert from Sydney Business Insights - Dr Sandra Peter. So Sandra Peter what's up with this?
Sandra: So this story comes out as yet another one of these companies has actually come to Sydney. Mobike launched in Sydney yesterday. And it's just the latest one to join companies like Obike, Ofo and Reddy Go who are already in Sydney. There is a number of ways these companies could be making money and the most intuitive one is actually not the one that's generating them funds at the moment. The most intuitive one is the dollar that people pay for 30 minutes of riding the bike and you'd think with these companies having something like 100 million users in places like China that would be a great source of revenue. Turns out it's not. In a previous podcast we did on bike sharing we talked about a study that NACTO the National Association of City Transportation Officials had done in the US and they were looking at how much you would need to use these bikes to return a profit. What they suggested was that you need more than four rides per bike per day to actually make money. Unfortunately in most major US cities these bikes are usually used between zero and two times at most and even in cities like New York where arguably people really really use bikes, it's still less than four rides a day. So that's not a good way to make money.
Kai: So we also talked on the previous podcast about the problems that these bikes create in inner city environments where people leave these bikes in public places, they're scattered on lawns in parks, there's been a number of bikes being thrown into the Yarra River in Melbourne and so they've become a bit of a nuisance which creates a bit of a problem especially for people who are in favour of bikes and bike-sharing. Here is Richard Glover on Thank God It's Friday making the point quite succinctly.
Audio (Richard Glover): We're all in favour of cycling right. We're also against the commercial operators who take over public space to run a business. What to do? Not since that first person tried to have his cake whilst simultaneously eating it has there been a dilemma this exquisite.
Kai: So the dilemma is really that on the one hand we should be very happy for bike-share companies to come to our cities and encourage the usage of bikes especially given how congested our cities are. But at the same time having the bikes around in public places can become a bit of a nuisance. So this is a problem to be solved. But that's not entirely what we're going to talk about. So how are those companies making money if not from the rental fees.
Sandra: Another source of revenue for these companies is actually charging security deposits, these start-up fees. So when you joined the app, in order to get access to the bikes you will pay something like $69 deposit for something like Obike or the $99 membership fee that you pay for Reddy Go. Now imagine some of these companies, companies like Mobike in China have over 100 million registered users for something like $99 or whatever it is they charge in China.
Kai: Yeah even if they charge considerably less at these numbers they have real money in the bank that they can use so there's a financing side of generating an income.
Sandra: So this is money that they can hang on to, that they can invest, that they can leverage in various ways.
Kai: But in the article you highlight a much more interesting way to look at these bike-share companies and that is data.
Sandra: Every time you pick up one of these the app and the bike generates data around where you've picked it up, what time, where you've ridden it, exactly what route you have taken, when you chose to drop it off, how soon you've decided to take another one and so on.
Kai: Also how fast you were going, which intersection you had to wait for how long so all of this data is aggregated by the bike-share company.
Sandra: And there are interesting ways in which you can reuse this stuff right. You could use it to target advertising for instance for the people who are using it. You could combine it with other datasets. For instance one of the big investors in some of these bike-share companies are companies like Tencent that already have a lot of data from other sources. Or you could sell it to third party companies and make money that way.
Kai: So let's explore this idea of aggregating and selling and using the data a little bit further. So we'll take a look at another app called Strava which is targeted at runners and bikers who run and bike for fitness purposes so people in lycra that you see on a Saturday morning riding in groups across the city, out into the country, they can use Strava to track their right, every one of them has Strava on their phones, they have their phones handy. And so they create a social experience out of riding. You go for a jog you take your Strava on your phone, it tracks and charts your run on a map and it also then combines that with a social experience where if you run around the lake say and that lake is being used by other people and someone has actually created a so-called segment then you can compete against other people around that lake and you can see your name on a leaderboard. And so you have this whole idea of a social fitness or social riding app. But every time you do this and anyone who does that will create data points that the company then aggregates.
Sandra: How does it use that data?
Kai: So Strava uses the data it generates from cycling in three different ways and they're very upfront about this. They have three different websites or products they called Strava Laps, Strava Insights and Strava Metro. Strava Metro is the product that is targeted at city councils and transportation departments. They can show those councils where bike traffic is happening, at what times, what wait times they have. And so those councils and transportation departments can then use that data in city planning to potentially put in place bike lanes and infrastructure.
Sandra: Hang on a minute. Wouldn't this be a bit of a problem. Isn't it that only a certain type of people get in lycra and cycle around lakes on Saturday mornings or Sunday afternoons?
Kai: Absolutely. And while don't it's not the only use for the app, a lot of people use it when commuting to work, you get quite a skewed view of your activity around the city and there's actually an article in CityLab a few days ago where that problem was actually highlighted. So it was said that yes it's a great idea to do this but city councils are quite aware that those apps are usually used in more affluent areas and also for certain users and also only about 10 percent of bike rides are actually captured using those apps. So sure you have to be very careful that you don't base your decisions solely on this biased data. But it is one important data point in a whole range of data that you can use, in Sydney for example you can combine that with Opal data, you can use eTag data, toll data from your highways and bridges. So you have all these different data points. And my point is that on top of the Strava data, if the bike-share companies provide further data points, we're getting a more and more complete picture about bike traffic in the city which can then be used to decide where to put in bike lanes, where to build our infrastructure, where our traffic lights might have to be changed.
Sandra: But let's highlight that some of the problems we have with the Strava data, we will also have with the bike-sharing companies. So Mobike for instance has only launched in Green Square. It's very unlikely that these companies at least in the first instance will move to let's say a low density area if you live somewhere far away from the city centre or areas that are less affluent where maybe less people can afford this because let's remember for a lot of these bike sharing companies the model doesn't get cheaper the more often you ride which is what happens usually with public transport, the more you ride it, the cheaper it gets but rather stays at the same price so it's less likely that companies will enter those areas especially if as in Sydney we're seeing an increasing competition with four or five companies coming in where prices might have to drop further so companies will have less resources to relocate those bikes or take them outside of city centres.
Kai: And it also raises the question, if those companies are allowed to put their bikes in public places and use public infrastructure, why should then councils and transportation departments have to pay for that data. Couldn't there be a mutual agreement where data is being exchanged for access to public spaces and wouldn't that alleviate some of the concerns around usage of public places?
Sandra: And you could argue that that mutual agreement is already in place. Waverley's Mayor John Wakefield (Waverley is a suburb of Sydney) said that at the moment they're cleaning up the bikes for free which each bike costing about 50 bucks to relocate so that's quite a cost to clean up these bikes. We might argue however that all these bike-sharing schemes are still in fairly early stages even though some of them had really tremendous investments for instance companies like Mobike have had 930 million dollars poured into the company. So they're still in early stages so they still have some time to figure out what the business model is whether that is fees or data and data at this point is looking as the most promising way. And there's still many ways in which they can experiment with where they want to take this.
Kai: And indeed that is what Strava is also doing and they have been on the market for far longer. So the other two products that I haven't mentioned yet is Strava Laps and Strava Insights so Strava Insights is a website where they let the general public, just anyone, play around with the aggregated travel data so you can basically zoom into the area in which you live and you get these heat maps and you can see where people are going, what are popular cycling routes and all of these kind of things. And then they have Strava Laps which is essentially a collection of engineering and research projects that they undertake themselves where they showcase what they are doing with the data and what possible uses the data might have. And it's a good evidence that they're still experimenting where to go with this. So for example they do trend analysis, analysis over time, they map data in different ways, they aggregate data, and what it shows is that this is in its infancy but that it has great promise for research, for understanding consumer choices, transport patterns and especially as we are talking about big changes to transportation moving forward moving to self driving cars, moving to ride share, and bike share. So the whole transport business is very much in flux at the moment and so decision makers and public planners will have to make evidence-based decisions in creating new and changing our city infrastructures. And so we think that any of those data will help but it will be a matter of combining them in the right way and overcoming the fragmentation that we see at the moment and gaining access to the data which potentially those companies want to exploit as a source of income.
Sandra: I for one am quite keen for this to work out. I moved here from the Netherlands. I ride bikes a lot. I would love to see better infrastructure and I think one of the keys for getting better infrastructure is to actually have more people biking hence highlight the need for better bike lanes.
Kai: Yeah Sydney is so bad. I mean at the moment riding a bike is just not for the faint-hearted right. So if those bikeshare companies are putting more bikes in and actually getting people to ride will lead to a rethinking of public spaces to put more bike lanes in, if that is the impetus needs to overcome the chicken and egg problem, that actually might be a good thing. But also let's not forget that those bikes are only in high density areas and the companies are very explicit about this because it doesn't pay off otherwise we need to make sure that the creation of bike lanes and infrastructure extends beyond the network that is covered by those bike-share companies and what potentially shows up in the data.
Sandra: So while cycling will for sure make you healthier, the jury is still out on Watson. Our second story for today.
Kai: So IBM Watson, the artificial intelligence program that famously won Jeopardy back in the day, has evolved into products that IBM has taken into multiple different areas, most famously into the health sector.
Sandra: So this is about Watson for Oncology, the AI that supposedly fights cancer. And the article we're discussing is from Boing Boing and it's by Cory Doctorow.
Kai: Cory Doctorow is a science fiction writer, author but also a blogger and science writer.
Sandra: And he's reporting actually on the article that broke this story.
Kai: The article in Statnews which reported in September that IBM pitched its Watson super computer as a revolution in cancer care but that it's nowhere close and that reality unfortunately is not matching up with the hype.
Sandra: So let's first look at what this was suppose to do and what it's actually doing. So about five years ago IBM announced that the Jeopardy winning Watson would now revolutionise cancer treatment and the way it was going to do this was to basically use artificial intelligence to ingest and digest and analyse thousands and thousands of studies, of research papers, of oncology articles that are published every year, in addition to a lot of patient level data that it would again ingest and a lot of expert recommendations and these would all somehow magically boil into a treatment recommendation. And the article published by Statnews and picked up by Cory Doctorow actually shows you well that's actually not what happens.
Kai: So while is indeed this machine learning component that ingests all that data, obviously the algorithm has to be trained constantly. So IBM teamed up with experts from Memorial Sloan Kettering Cancer Centre in New York to basically run Watson for Oncology the AI based service. And this service this Watson for Oncology service has since been sold to around 50 hospitals in the US and worldwide. In India, South Korea, Slovakia all over the world where it's being used. And the authors make the point though and it's expressed in the title of the article that Watson for Oncology isn't an AI that fights cancer, it's an unproven mechanical turk that represents the guesses of a small group of doctors. So the point that they're making is that while doctors in these clinics that use Watson can submit their patient records and their patient data for Watson to consider and come up with recommendations...
Sandra: The treatment recommendations are not actually based on insights that the algorithm would derive from these data but rather it's based exclusively on training that the algorithm received from human operator. There's a couple of dozen physicians at this one hospital who told Watson how patients with specific characteristics should be treated.
Kai: Which not surprisingly comes with certain problems and one of the main problems that the authors highlight is one of bias. So not surprisingly and we've discussed this before any AI, any machine learning that is trained from one perspective by one group of people with a certain view of the data will always espouse the biases of that perspective that was put into the algorithm and in this case the authors make the argument that in many cases the recommendations that are coming from this hospital were not matched with the reality of the health systems for example in which those treatments have to be provided. There's issues with certain treatments being recommended that are not covered by local insurance schemes, that do not even match the biology of Asian patients that have issues with the fact that the hospital in New York serves a particular demographic. So there's all kinds of problems with this.
Sandra: And we do want to acknowledge the fact that intuitively it still makes sense right. Who better to train this AI than the best doctors at the best cancer centre in the world but cancer treatment is actually not that simple. So yes you recommend one course of treatment but that then fails; agreement of what the next best course of treatment would be varies widely between experts even experts in the field even experts at the same hospital. What could work next? What to try next when the chances are really low that something will work?
Kai: Yes and the problems of bias don't end there. Feeding the algorithm with articles and knowledge that it learns also represents certain choices that doctors are making.
Sandra: However when Watson gives you the evidence so when it makes a recommendation it also provides you the relevant studies and all the background information and so on to support this recommendation. But the recommendation itself is derived by these hospital doctors who've also curated some of the studies that are included in these databases.
Kai: Yes. And the experts at Memorial Sloan Kettering and IBM have come forward and said sure this is biased but it's an unapologetic form of bias because we have the best experts working on this and they're putting their knowledge in and what can be better than having their views represented here. And while that is true and while it is certainly commendable that IBM has teamed up with one of the best cancer centres the authors in the Stats article have gone around and done a lot of interviews with people in different countries and they find that certainly the background information that the Watson algorithm provides is sometimes useful in backing up a decision and that in many cases the recommendation that Watson would come up with is in line with what the local doctors would have recommended.
Sandra: However that's not always the case. So for instance the article refers to researchers in Denmark and the Netherlands who said that hospitals in those countries had actually not signed up to Watson because it seems to have a very limited view on international literature stressing too much American studies and adding very little in terms of international or European or other parts of the world.
Kai: And this is certainly a problem that many colleagues in different academic fields can relate to. In my discipline for example the publication process is very much geared towards publishing in North American journals and when you come with a European perspective you always have to justify why this is special and not like the American perspective so there's always these tendencies that there's cultural biases in scientific data and that it reflects some reality better than others. So there's a problem there. But the main problem with the bias is not that the initial data has this American perspective but that the algorithm doesn't actually learn from what is happening by the treatments being used in various jurisdictions because there's no feedback, there's no learning process.
Sandra: Which brings us to the second issue raised by these articles which is it's not only that there is a bias that you could solve by having a feedback mechanism but that there actually is no such feedback mechanism.
Kai: Yes so you could take the view that Watson is relatively young right so it's toddler age so give it some time. Doctors learn for years and years to build up expertise so we should allow Watson sufficient time to learn and become a real expert and then outsmart doctors in the process because it can digest so much more information. So that's one of the promises.
Sandra: But at this time if let's say I am a doctor somewhere in Slovakia and I employ one of the recommendations and then I see a negative outcome or I see a benefit by employing something that the algorithm hasn't suggested to me that will never actually go back and be reintroduced in what Watson knows.
Kai: And we don't say that it couldn't be fed back into the algorithm, we're saying that at this point in time it's not and the algorithm is solely learning from the experts in New York. So there is no feedback loop from the various hospitals in which decisions are being made or supported with Watson.
Sandra: And this is unlike a lot of other AI systems that we see, things like what Amazon employs. So when Amazon uses AI to make recommendations or change how the pages are laid out or the navigation process and so on, all the time measures sales, measures engagement and figures out whether that actually produced the best outcome, the outcome that they wanted or not, so it's able to learn from that. And Cathy O'Neil in Weapons of Math Destruction actually discusses the real dangers associated with algorithms and AI that do not have feedback mechanisms built in. And she talks for instance about evaluating teachers performance and firing teachers based on subpar performance of kids on standardised tests and so on and so forth.
Kai: And there's a third problem which is the fact that IBM has been very optimistic in claiming that Watson would be fed with lots of patient records, patient data, medical records but it turns out that this is not easy.
Sandra: Not only not easy but actually truly challenging. Think about medical records. There are a lot of acronyms in there. There's a lot of shorthand phrases, there are human errors sometimes when this data gets inputted. There are different styles of writing. Again if you think about what patient charts would look like at a New York hospital they're probably quite different to a patient chart in Mongolia for instance. So teaching the machine to read these patient records actually prove to be a lot harder than anyone at IBM thought in the first instance so people are spending a lot of hours trying to get this deeper into the system to begin with.
Kai: And at the heart of this is a technical issue with machine learning that we haven't really sufficiently highlighted on the podcast before which is the data labelling issue. Whenever we input training data into an algorithm we need to tell the algorithm pretty clearly what the various bits of data are about, whether they are related to the diagnosis part or to the medical information part, the patient record part. So in other words anything that we feed into the algorithm we actually have to label for the algorithm which is not only hugely laborious is also sometimes really hard because hospitals have different ways in which they keep medical records, different jurisdictions have different conventions about what kind of information are being kept.
Sandra: And also there are huge costs associated with this so someone actually has to pay and make an investment into getting all this data online. This is not part of what doctors or nurses or administrative staff are paid to do.
Kai: So it turns out for IBM in making Watson work they are tapping right into the large unresolved issues around standardisation of medical records. And this has been an ongoing topic in the health sector for a decade at least. The idea to create a standardised electronic patient record which is still not reality in most jurisdictions.
Sandra: So what's a way forward? What's a way out of this mess? Because Watson is one of those things that we really wanted to work and wanted to work well because a) it already has some fantastic benefits in places like Mongolia where you have a hospital in the capital of Mongolia that has zero oncology specialists where this system modified in some ways would bring great advances. And we do think that artificial intelligence actually has the potential to change fields like medicine or a cancer treatment or oncology. This would be fantastic. So we want this to succeed.
Kai: And from a technical point of view I think it is quite significant that Watson's machine learning engine is able to match the recommendations of cancer specialists in many cases so there's only very few studies and very few stats around this but we've seen some data from India for example where the AI achieved a concordance rate of 96 percent for lung and 81 percent for colon and 93 percent for rectal cancer. So these are reasonably good stats and while those stats are not necessarily matched in other countries such as South Korea where the percentages were much lower, it shows that machine learning can actually in some way replicate what cancer specialists can do.
Sandra: But going forward we do need to build in those feedback mechanisms. Just think of a situation where you would be a young doctor who has access to the data that Watson has and let's say I disagree with a older very experienced oncologist and he would say this is the course of treatment I would say no but my opinion is supported by Watson and 20 or 30 different studies. You might be likely to fold and we will go with my suggestions even though you might be experienced, you might have local knowledge, understand the context better, understand the patient better. So we need to make sure that the way this is brought in to real life is actually responsible.
Kai: Which brings us to the actual story here. So this is not a story about failure of a AI because we do believe that there is real benefit in using machine learning here. This is really a story about the failures of the IBM marketing machine who have basically sold Watson as a magic miracle machine that can at a touch of a button cure or in their lingo solve cancer. And this is also the point that these articles are making, they're not saying that this program is a failure or that machine learning won't have any significant impact on how we might organise cancer care but that the expectations are vastly overblown, that the hype is completely out of line with reality and that this is doing the whole project of machine learning in health care a huge disservice potentially. So let's unpack this.
Sandra: So very quickly there there's a huge danger in raising expectations about what this might do.
Kai: So one of the biggest claims that IBM has made is that Watson will be able to look for patterns and derive treatment recommendations that human doctors might otherwise not be able to come up with because Watson is so much better at digesting huge amounts of data and by having this artificial intelligence it would be able to combine that data and to come up with entirely new forms of cancer treatment.
Sandra: Not happening at the moment.
Kai: No it is not happening and the article goes to great lengths making the argument that this is really problematic in principle. And one of the reasons is that whenever we train an algorithm we actually have to tell the algorithm what it's looking at, so we have to label the data and we mentioned that. But that means that any data that we label will already have to be understood by experts so anything that we input we already understand. And so the algorithm is unlikely to find anything new by merely recombining what we already know.
Sandra: And recombining it along rules that we give it.
Kai: Absolutely. And so the idea of say AlphaGo which comes up with entirely new ways of playing Go doesn't actually work here because it's not that kind of machine learning, it's machine learning that relies on past training data. So IBM is vastly overclaiming what Watson can do and therefore raising expectations that the system cannot possibly fulfil at least not in its current incarnation.
Sandra: There's also nothing published in peer reviewed journals at the moment. So far anything that's come out of Watson has come in the form of conference abstracts and all of these studies, every one of them, was either conducted by a paying customer of IBM Watson or included IBM staff on the author list. Or even both of these things.
Kai: So the article makes the point that IBM is not subjecting Watson for Oncology to a rigorous scientific assessment. They are selling this as a commercial product but we do not have any data or evidence that will give us any indication of its real performance. And the authors assert that IBM might be resisting doing these kinds of evaluations because they might not be interested in what they might find given what they have claimed Watson will be able to do and what it is actually capable of doing at present.
Sandra: So the fact that IBM is not being exposed to a review by outside scientists or conducting clinical trials is actually a really murky area because Watson is a product that is not under development, it's currently deployed but still being tested. Even though it's not uncommon for companies to avoid review very early on, this product is already in hospital and people are already using the system to either validate or get a second opinion on what they're doing. The really bad news we think however for IBM is the fact that if we have such a hype product that promises so much and then there is a huge backlash when we realise it is actually more along the lines of a mechanical turk rather than real artificial intelligence, people will resist using it and improving it and companies like IBM might actually refrain from investing the huge amounts of money we need to actually make this work. Think about the fact that IBM had to spend 11 and a half times its annual earnings to develop something like the mainframe computer. Curing cancer in equivalent terms through IBM Watson would require IBM to spend something like one hundred thirty seven billion dollars to do this.
Kai: And they're not even close. And lets not forget that the mainframe has been the cash cow for IBM for decades and given that they see Watson for healthcare as the next big thing on which the company fortunes will ride there is no evidence at this point that they are actually putting the required financial backing behind this or so the authors say. And so at the moment the reality of what Watson is doing and what Watson is and the hype that the IBM marketing machine has created is show a vast discrepancy to the point that Oren Etzioni who we've cited before - the CEO of the Allen Institute for AI - says "IBM Watson is the Donald Trump of the AI industry. Outlandish claims that aren't backed by credible data. Everyone, journalists included know, that the emperor has no clothes but most are reluctant to say so." So that's not a really good assessment. So the main problem here is not that machine learning might not be useful in healthcare or that Watson might not grow into something really valuable but at this point in time the chasm between the hype and the expectations and the actual delivery is such that it might actually put the whole idea of machine learning in healthcare in disrepute and let's not forget the ethical issues because we are playing with the hopes of people and patients we want to quote one of the designers whose previously worked on the Watson product who walked around the hospital and was approached by patients who said that they were waiting for Watson to come online because they had put great hope in the capabilities of finding new cures for cancer and that the designer said that was actually pretty heartbreaking for me as a designer because I had seen what Watson for Oncology really is and I was very painfully aware of its limitations.
Sandra: So what we're hoping is that cases like this don't actually lead us down the slope of disillusionment where we give up investing in AI and give up trying and actually do not realise the full potential of some of these technologies.
Kai: Just because we weren't able to fulfil in the short term I think in the long term this will work but it needs way more time and it's much more difficult than IBM had us believe.
Sandra: So Kai, I have no idea how to go from this story to our last story which involves buying makeup on Spotify.
Kai: Well I believe you just did. So that story is really an update on the business of Spotify which we've discussed on the podcast previously. So for those few of you who don't know Spotify is a music streaming service that you can sign up for and then for a monthly fee listen to the almost entire catalogue of world music on your devices, computer, car dashboard, phones, online.
Sandra: The story comes from Vanity Fair and reports on the fact that Spotify has added a new maybe surprising service which allows you to basically buy beauty products, things like lipstick or eyeliner, directly through Spotify.
Kai: So Spotify now sells beauty products but it's not actually Spotify doing this. It is Spotify opening up its platform for musicians to sell products directly to their followers, to their fans, to the music audience, so on top of the ability to just stream music we can now purchase merchandise, beauty products, concert tickets, directly from musicians and apparently Spotify is not taking any commission, it's not taking any cut out of those purchases. So what is this all about? This doesn't seem to be Spotify trying to make more money.
Sandra: Spotify has for a very long time been accused of the fact that other than musicians who are really really famous and whose songs are played a lot nobody else can actually make money out of Spotify. So the musicians with fewer play actually really struggle to make money from music in this model.
Kai: And there's a number of articles and we put a couple in the shownotes where musicians have actually put their data on the table and showed that even if you have a million listens on Spotify that might only translate into a few thousand dollars in royalties so except for the most famous and well known artists, musicians will find it hard to make a living just off the royalties they draw from Spotify or for that matter similar other streaming platforms.
Sandra: And this is why our podcast will always be free.
Kai: Yes. Sandra and I have done a back of an envelope calculation and based on the listens we get on the podcast which are not bad and they're increasing and by the way tell all your friends about the podcast but even then if we received half a cent per listen which is basically the going rate on Spotify it wouldn't be enough for us to have a coffee together per episode which just goes to show how hard it will be for these artists to make a living off Spotify.
Sandra: The article in Vanity Fair and similar articles which have cropped up in Techcrunch and in the Verge and so on actually say that this is a move by Spotify to sweeten the deal for all of these artists who are not making a living out of Spotify.
Kai: And we've also previously mentioned that for most artists it's no longer about making money from albums selling music or this streaming. The money is now in giving concerts and selling merchandise so all of the kind of things that surround the actual core music. And so this is a way for Spotify to open its channel for that side of the business for artists actually make a living off selling things. But that has also given us pause.
Sandra: So it turns out this is actually counter intuitive because the only people who would be able to make money out of let's say selling lipstick or eyeliner or other merchandising products are the artists who are very popular to begin with, who actually might already be making money out of Spotify. So if I'm an upcoming artist with a very small followership, how am I going to be able to release all these merchandising products and who exactly is going to buy it?
Kai: Yeah. And in the case mentioned in the article there's a famous makeup designer who teams up with artists to create their makeup line. If you're an up and coming artist you're in no position to actually strike these kinds of deals.
Sandra: So while we want to give points to Spotify for actually not making this a revenue stream and taking no cut whatsoever from the sales that happened through their platforms, so well done them.
Kai: The actual story is that this is a glimpse into the future of these streaming platforms to become more fully fledged platforms for artists to engage with their audiences, beyond just listening to music and actually monetising these streams and maybe this is just the beginning and there will be other ways in which artists can engage and indeed make money even when they're not in a position to create their own beauty line.
Sandra: So just the beginning for them, probably the end for us because that's all we have time for today.
Kai: That's been a long podcast. Thanks for listening.
Sandra: Thanks for listening.
Outro: This was The Future, This Week made awesome by the Sydney Business Insights team and members of the Digital Disruption Research Group. And every week right here with us our sound editor Megan Wedge who makes us sound good and keeps us honest. Our theme music was composed and played live from a set of garden hoses by Linsey Pollak. You can subscribe to this podcast on iTunes, SoundCloud, Stitcher or wherever you get your podcasts. You can follow us online on Flipboard, Twitter, or sbi.sydney.edu.au. If you have any news that you want us to discuss please send them to sbi@sydney.edu.au.
Close transcript