Ben Fahimnia and Elton Brown
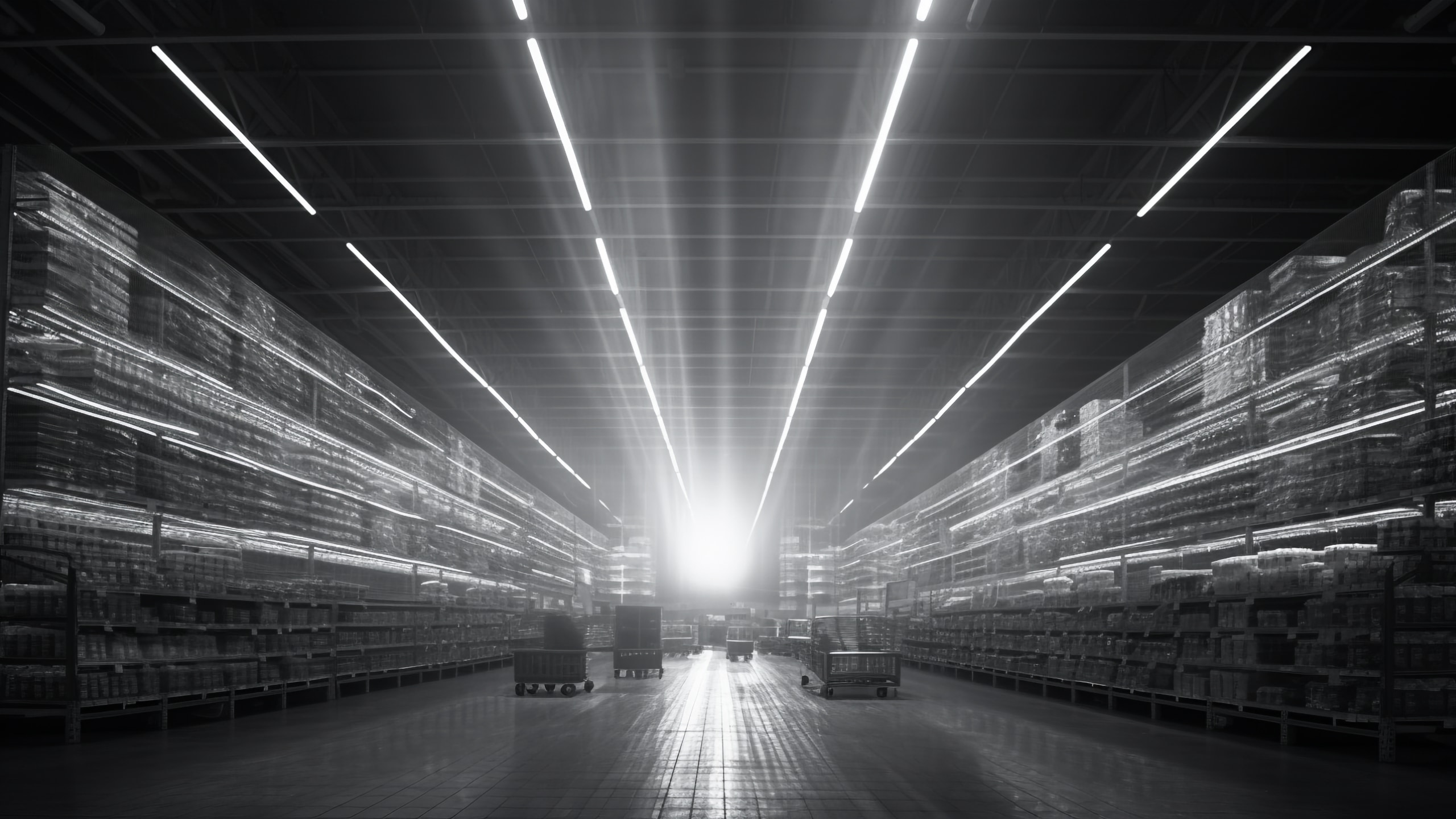
Dial AI for assistance: leveraging AI for supply chain resilience and crisis management
Imagine a plotline in a movie thriller (corporate genre):
Artificial intelligence (AI) capabilities save a food supplier encountering a crisis after their largest supplier abruptly goes out of business due to a complex web of unethical operations, geopolitical conflicts, and local regulations.
AI saves the day against impossible odds and villainous characters. But this is a ‘real life’ supply chain disruption scenario in which we were involved, and an AI did ‘come to the rescue’ with a nuanced solution that saved the manufacturer time and money.
But in too many scenarios, AI is presented as almost magical thinking that can be sprinkled over any problem and – hey presto – the AI autonomously comes up with a solution.
Like a detective in the movie’s final scene, this article aims to explain how the AI’s exceptional powers of calculation actually get to work on a problem.
Scene one: the unexpected crisis
Faced with the risk of losing market share and decades of brand loyalty, a beloved Aussie king of convenience snacks swiftly convenes a cross-functional crisis meeting to find a solution and save their business. Leveraging the power of AI and machine learning, they embark on a journey of supply chain optimisation to navigate the crisis and secure their customer commitments.
In the world of business, unexpected disruptions can pose significant challenges to even the most successful companies. The Australian food manufacturer in question had achieved long-term success driven by brand loyalty and market growth. However, their prosperity faced a formidable challenge when their largest supplier, based in Asia, unexpectedly ceased operations overnight due to external factors beyond their control.
With supplier lead times standing at eight weeks, and only ten weeks of ingredient cover in the local warehouse and enroute from the supplier, the company faced the risk of running out of stock for 70% of their product range by week eleven. Customer orders were already committed for the next six weeks, and sales forecasts projected a seasonal demand peak for the quarter, intensifying the urgency to find a viable solution.
Scene two: in the disaster response meeting
In a bid to address the crisis and maintain their market position, potential solutions are explored during the cross-functional crisis meeting. Recognising the complexity of the situation and the need for data-driven decisions, the team immediately utilises their new AI-driven supply chain optimisation platform, developed by a specialist supply chain technology vendor, to evaluate multiple disruption and mitigation scenarios, considering costs, margins, revenues, service levels, and risks across the extended supply chain.
Different variations of the following scenarios were evaluated by the AI-driven optimisation model to find the optimal solution that aligned with their business objectives:
- Component substitution: utilising alternative ingredients at a higher cost to replace the unavailable ones.
- Product substitution: providing customers with alternate products, potentially leading to a margin loss.
- Local sourcing: turning to local suppliers, even at a higher cost, to secure ingredients in the short term.
- Local production: opting to manufacture the ingredients locally, instead of relying on external sources.
- Demand shaping: changing customer prices to influence demand up or down.
- Cancelling customer orders: a last resort that would result in losing sales but could be necessary in extreme situations.
Through the integration of AI and machine learning, the platform performed millions of complex calculations in a matter of seconds. The team reviewed advanced analytics and adjusted business policies and parameters to refine the scenarios, ultimately leading to the identification of the optimal solution.
The recommended scenario proved to be a sophisticated blend of all alternatives, strategically applied across various ingredients and finished goods. The solution accounted for multiple suppliers, both local and international, and considered pricing and competitor activities.
Scene three: the plan succeeds and our company triumphs
Within a remarkably short time frame, the IBP (Integrated Business Planning) team reviews and approves the recommended scenario. The revised sales forecast, inventory targets, production plan, purchasing plan, and pricing strategy are swiftly integrated with the central transaction system (ERP). As a result, the company gains a significant competitive advantage over competitors who might have faltered following such a substantial supplier shutdown.
The case study exemplifies the importance of leveraging AI in times of crisis to drive supply chain resilience and secure business continuity. Through the innovative use of technology, the Australian food manufacturer not only averted potential disaster but also emerged with a revised strategy that aligned with their business goals.
This successful adaptation underscores the transformative potential of AI-driven analytics in guiding businesses towards data-informed decisions, ensuring their ability to weather unforeseen challenges and thrive in an ever-changing marketplace.
Image: generated in Midjourney using a paid plan within their Terms of Service.
Ben is Professor and Chair in Supply Chain Management at the University of Sydney Business School as well as Director for Supply Chain & Logistics Association of Australia and the Founding Director of Sound of Silence Productions Pty Ltd. His research interests include supply chain transformation and behavioural decision-making.
Elton Brown is a Senior Consultant at Demand Management Systems. He is also an Adjunct Lecturer in Intelligent Supply Chains at the University of Sydney.
Share
We believe in open and honest access to knowledge. We use a Creative Commons Attribution NoDerivatives licence for our articles and podcasts, so you can republish them for free, online or in print.