Sydney Business Insights
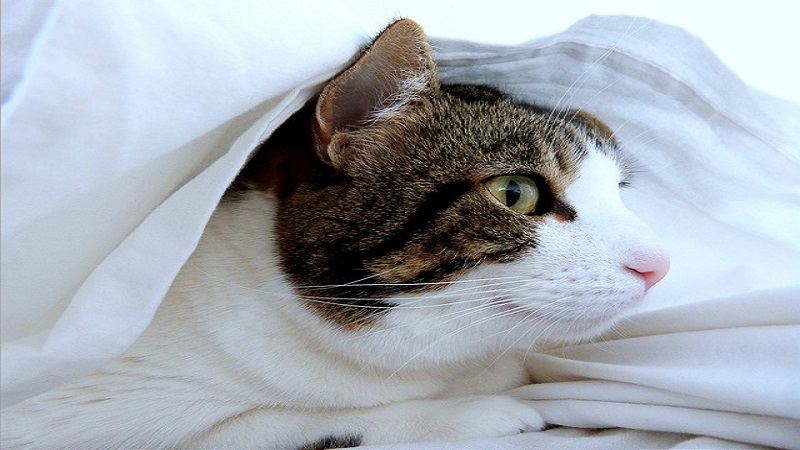
AI can’t see my cat
Japanese and MIT researchers have been finding flaws in image recognition systems that are based on AI machine learning algorithms. These algorithms, known as ‘deep neural networks’, learn, by being trained with lots of sample pictures, to recognise particular objects – say turtles, cats, dogs – and importantly to recognise how these subjects differ.
Google and Facebook use such image recognition algorithms in their platforms to categorise images posted by its users, for example to then place relevant ads next to them.
The BBC reported that MIT and Kyushu University researchers created ‘adversarial examples’ to test the how well these image recognition algorithms work. The aim of these adversarial examples was to fool the algorithm into seeing what is not there.
By changing even sometimes just one pixel in the image the AI misidentified the object in every instance tested.
A series of doctored turtle images were recognised as a rifle by the AI. A photo of a cat was identified as a bowl of guacamole, second guess broccoli, third mortar – so not even close to recognising it as a cat.
These are not mistakes a human would make – to us the ‘altered’ image is still clearly a turtle, cat etc. So, how big a problem could this be? Think of the role of a machine vision algorithm working in a CCTV camera or an autonomous vehicle.
Listen to Sandra and Kai’s discussion on The Future This Week podcast @00.35
While no one has claimed machine learning algorithms will be 100% accurate they have been shown to be better than humans in certain tasks, such as finding cancer cells in MRI pictures.
This latest research demonstrates the general weakness in these kinds of algorithms and potentially a way to attack and maliciously exploit visual recognition systems. And while the algorithm in this turtle/gun example was publically available and therefore easy to play around with, the authors also mention that they are working on techniques to ‘attack’ less accessible systems.
These studies also debunk the assumption that algorithms learn like humans. It becomes clear that distinctions between particular objects are not made in the same way that humans categorise – we would not group turtles and rifles or cats and guacamole in any way close together. The reason is that the algorithms merely identifies pixel patterns without any understanding of the objects itself.
So while algorithms can do amazing jobs we must appreciate they are not infallible and of more concern – they are also fragile in that they are vulnerable to these kinds of attack.
What if an algorithm in a CCTV that was supposed to detect a gun was deliberately fooled into thinking it was a turtle?
And while Google and Facebook are working on building algorithms that will be more robust in the face of malicious attacks – we might be about to enter an algorithmic arms race where the algorithms are getting better but so too are the means to fool them.
To hear Sandra and Kai discussing these stories, tune into this episode of The Future, This Week.
Sydney Business Insights is a University of Sydney Business School initiative aiming to provide the business community and public, including our students, alumni and partners with a deeper understanding of major issues and trends around the future of business.
Share
We believe in open and honest access to knowledge. We use a Creative Commons Attribution NoDerivatives licence for our articles and podcasts, so you can republish them for free, online or in print.