Sydney Business Insights
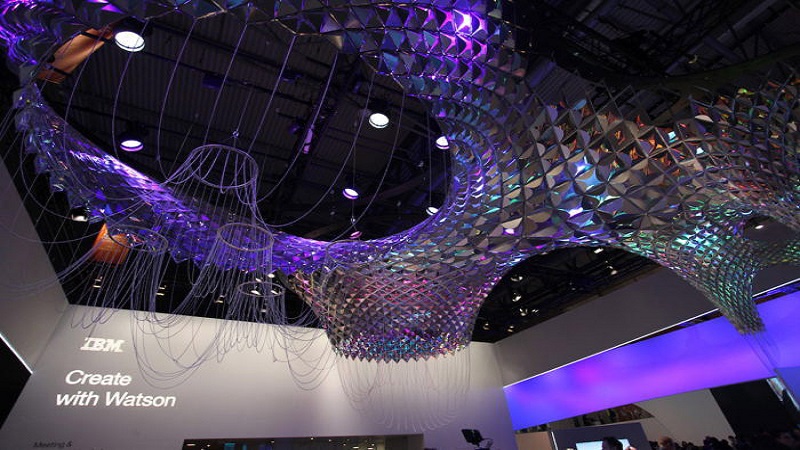
Watson’s health problems
Six years ago IBM’s AI ‘supercomputer’ Watson beat the two all-time best players in Jeopardy! One of the defeated contestants ceded his crown willingly with the now infamous phrase “I, for one, welcome our new computer overlords.”
From game show triumph, IBM announced Watson’s next task was to revolutionise cancer treatment. Today Watson for Oncology (WfO) is at play in 50 hospitals on 5 continents where doctors input their cancer patient records and WfO makes treatment recommendations and suggests journal articles for further reading.
Watson’s promise of super human treatment is based on the premise it would use AI to ingest and analyse thousands of oncology studies, millions of patient records along with expert recommendations – and out would pop individual treatment recommendations.
However according to scientific and other reports Watson, far from delivering AI derived insights into cancer treatment, is no more than a ‘mechanical turk’ – a human driven engine that, while using artificial intelligence, relies heavily on human judgement.
The human component of Watson Oncology is provided by a small panel of cancer experts from New York’s prestigious Memorial Sloan Kettering Hospital (MSKH). Watson’s treatment recommendations are not derived from the algorithm but are based exclusively on training it receives from this select group of doctors.
Listen to Sandra and Kai’s discussion on The Future, This Week podcast @13.56
And this brings us to its first limitation: Bias.
Any AI that is trained from one group of people with a certain view of the data will naturally espouse the biases of that perspective.
Crucially the reports interviewed doctors in other hospitals who said WfO’s recommendations were often not suitable for their health systems or for their patients who were ethnically and socially different from the Memorial Sloan Kettering demographic.
Moreover the articles suggested by Watson also reflected a North American bias that European doctors considered offered a limited view of the international literature.
Second limitation: Watson has no feedback mechanism. Apart from the training it receives by doctors at MSKH, WfO does not take in information from any other hospital where Watson is operating meaning its algorithm is not learning and being improved via feedback on the outcome of the recommendations it provides. Contrast this with other commercial AI systems: Amazon’s AI makes recommendations for how its pages are laid out or how its navigation process works and it will take into account data from sales, engagement measurements that reflect any changes the AI suggested thus maximising the desired outcome.
The third problem: Labelling complexity. Medical records are complex documents with lots of acronyms, diverse writing styles, and cultural differences. All information inputted into an algorithm has to be labelled. And the labelling is not just a matter of naming conventions but telling the algorithm what the various bits of data are about – such as, is that piece of information related to the diagnosis part or the patient record part?
IBM underestimated how difficult it is to teach the system to read and analyse information when standardised electronic medical records are not even a thing in most jurisdictions.
It’s not that machine learning in the area of medical diagnosis is not a fantastic goal – how valuable would it be for a clinic in Mongolia be able to tap into the world’s best practice? But by over hyping Watson’s capabilities IBM is at risk of discrediting the notion of machine learning in health care and compromising the very ingredient it desperately needs – support from lots of medical practitioners to improve the AI and overcome its limitations so that it becomes a valuable tool that augments the work that doctors do.
To hear Sandra and Kai discussing this and other stories, tune into this episode of The Future, This Week.
Sydney Business Insights is a University of Sydney Business School initiative aiming to provide the business community and public, including our students, alumni and partners with a deeper understanding of major issues and trends around the future of business.
Share
We believe in open and honest access to knowledge. We use a Creative Commons Attribution NoDerivatives licence for our articles and podcasts, so you can republish them for free, online or in print.